|
ACCESS THE FULL ARTICLE
No SPIE Account? Create one
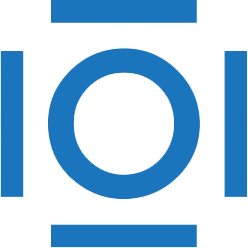
CITATIONS
Cited by 9 scholarly publications.
Detection and tracking algorithms
Target detection
Hyperspectral imaging
Hyperspectral target detection
Optical filters
Image sensors
Sensors