|
ACCESS THE FULL ARTICLE
No SPIE Account? Create one
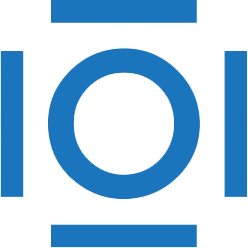
CITATIONS
Cited by 9 scholarly publications.
Error analysis
Sensors
Optical engineering
Statistical analysis
Detection and tracking algorithms
Image analysis
RGB color model