|
ACCESS THE FULL ARTICLE
No SPIE Account? Create one
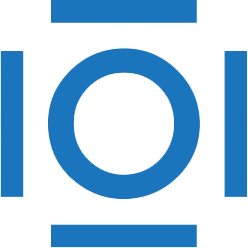
CITATIONS
Cited by 2 scholarly publications.
Visualization
Image processing
Visual process modeling
Image segmentation
Optical engineering
RGB color model
Data modeling