|
ACCESS THE FULL ARTICLE
No SPIE Account? Create one
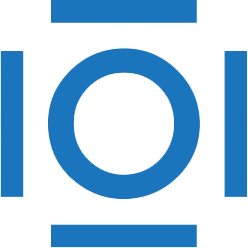
CITATIONS
Cited by 1 scholarly publication.
Robotics
Machine vision
Data modeling
Cameras
Image registration
Space robots
Visual process modeling