|
ACCESS THE FULL ARTICLE
No SPIE Account? Create one
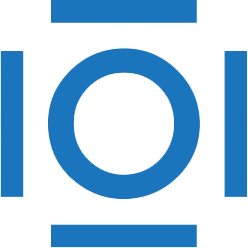
CITATIONS
Cited by 6 scholarly publications.
Image segmentation
Convolution
Neural networks
Image processing
Defect detection
Image classification
Classification systems