|
ACCESS THE FULL ARTICLE
No SPIE Account? Create one
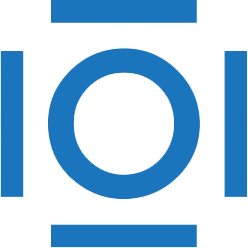
CITATIONS
Cited by 2 scholarly publications.
Synthetic aperture radar
Speckle
Image quality
Signal to noise ratio
Image filtering
Digital filtering
Image processing