|
ACCESS THE FULL ARTICLE
No SPIE Account? Create one
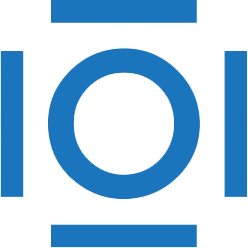
CITATIONS
Cited by 1 scholarly publication.
Image classification
Data analysis
Feature extraction
Analytical research
Statistical analysis
Machine learning
Shape analysis