|
ACCESS THE FULL ARTICLE
No SPIE Account? Create one
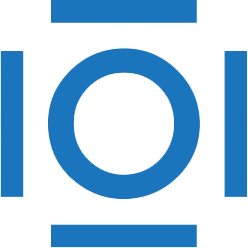
CITATIONS
Cited by 2 scholarly publications.
Target detection
Neural networks
Image filtering
Wavelets
Linear filtering
Detection and tracking algorithms
Particles