|
ACCESS THE FULL ARTICLE
No SPIE Account? Create one
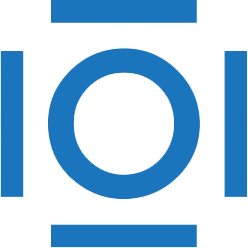
CITATIONS
Cited by 5 scholarly publications.
Neural networks
Feature extraction
Classification systems
Acoustics
Neurons
Data modeling
Autoregressive models