|
ACCESS THE FULL ARTICLE
No SPIE Account? Create one
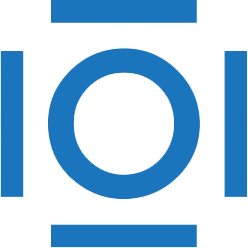
CITATIONS
Cited by 13 scholarly publications.
Motion models
Nonlinear filtering
Filtering (signal processing)
Systems modeling
Sensors
Electronic filtering
Stochastic processes