|
ACCESS THE FULL ARTICLE
No SPIE Account? Create one
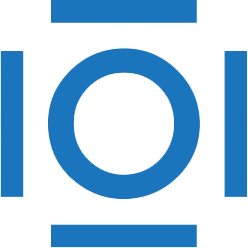
CITATIONS
Cited by 1 scholarly publication.
Image segmentation
Statistical analysis
Feature extraction
Defect detection
Statistical modeling
Error analysis
Algorithm development