|
ACCESS THE FULL ARTICLE
No SPIE Account? Create one
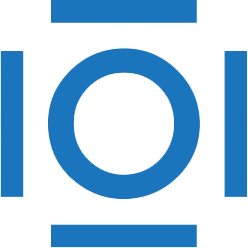
CITATIONS
Cited by 13 scholarly publications.
Particles
Sensors
Particle filters
Error analysis
Data modeling
Filtering (signal processing)
Nonlinear filtering