|
ACCESS THE FULL ARTICLE
No SPIE Account? Create one
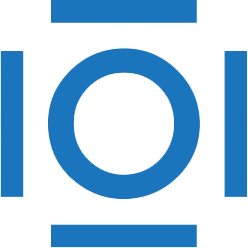
CITATIONS
Cited by 7 scholarly publications.
Sensors
Statistical analysis
Hyperspectral imaging
Detection and tracking algorithms
Target detection
Hyperspectral target detection
RGB color model