|
ACCESS THE FULL ARTICLE
No SPIE Account? Create one
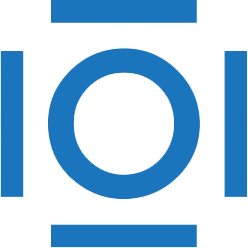
CITATIONS
Cited by 7 scholarly publications.
Principal component analysis
Independent component analysis
Minerals
Hyperspectral imaging
Remote sensing
Signal to noise ratio
Algorithm development