|
ACCESS THE FULL ARTICLE
No SPIE Account? Create one
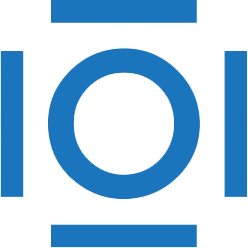
CITATIONS
Cited by 1 scholarly publication.
Principal component analysis
Automatic target recognition
Feature extraction
Image processing
Detection and tracking algorithms
Image filtering
Optimization (mathematics)