|
ACCESS THE FULL ARTICLE
No SPIE Account? Create one
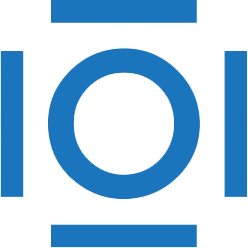
CITATIONS
Cited by 12 scholarly publications.
Detection and tracking algorithms
Target detection
Sensors
Algorithm development
Data modeling
Hyperspectral imaging
Statistical analysis