|
ACCESS THE FULL ARTICLE
No SPIE Account? Create one
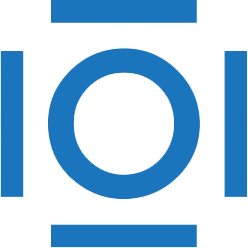
CITATIONS
Cited by 6 scholarly publications.
Target detection
Data modeling
Hyperspectral imaging
Clouds
Hyperspectral target detection
Detection and tracking algorithms
Image analysis