|
ACCESS THE FULL ARTICLE
No SPIE Account? Create one
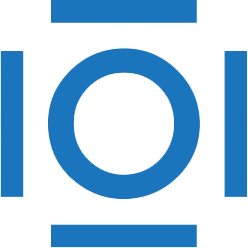
CITATIONS
Cited by 6 scholarly publications.
Target detection
Signal to noise ratio
Principal component analysis
Hyperspectral target detection
Hyperspectral imaging
Sensors
Detection and tracking algorithms