|
ACCESS THE FULL ARTICLE
No SPIE Account? Create one
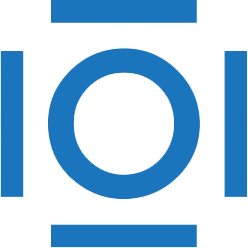
CITATIONS
Cited by 1 scholarly publication.
Ultrasonography
Image segmentation
Tissues
Ultrasonics
Speckle
Tumor growth modeling
Statistical modeling