A method for geometric distortion correction and space and time-varying blur reduction is proposed, which can recover the high-quality image from a single-frame image distorted by atmospheric turbulence. First, the U-net like deep-stacked autoencoder neural network model is proposed, which is composed of two deep convolutional autoencoder (CAE) neural networks and a U-net. The first CAE is used for feature extraction, the U-net is used for feature deconvolution, and the second CAE is used for image reconstruction. For the loss reduction of image information, transposed convolution instead of upsampling is selected in U-net networks. Moreover, in order to obtain sufficient feature information for reconstruction, the first CAE and the last CAE are symmetric skip connected. This not only enables the fusion of low-level and high-level information but also ensures the integrity of image information greatly. Then, a method of gradually mature training from simple to complex is proposed to overcome the difficulty of convergence on smaller training sets. It makes the network be converged and mature by increasing the complexity of training data gradually so as to restore the high turbulence-degraded image. Experimental results of actual observation data and simulation data show that the algorithm has a stronger antinoise ability and can recover image details and sharpen image edges more effectively. In particular, for atmospheric turbulence severely degraded image restoration, the peak signal-to-noise ratio index is increased by about 10% on average compared with state-of-the-art methods. |
ACCESS THE FULL ARTICLE
No SPIE Account? Create one
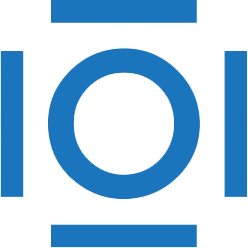
CITATIONS
Cited by 16 scholarly publications.
Image restoration
Atmospheric turbulence
Neural networks
Image processing
Visualization
Convolution
Signal to noise ratio