|
ACCESS THE FULL ARTICLE
No SPIE Account? Create one
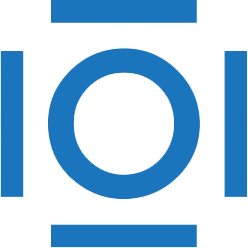
CITATIONS
Cited by 2 scholarly publications.
Video surveillance
Detection and tracking algorithms
Video
Image processing
Telecommunications
Video microscopy
Compressed sensing