|
ACCESS THE FULL ARTICLE
No SPIE Account? Create one
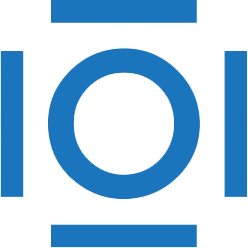
CITATIONS
Cited by 2 scholarly publications.
Feature extraction
Breast cancer
Image classification
Pathology
Medical imaging