|
ACCESS THE FULL ARTICLE
No SPIE Account? Create one
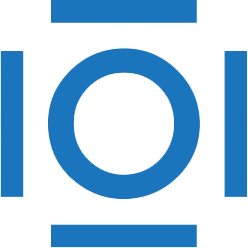
CITATIONS
Cited by 4 scholarly publications.
Statistical modeling
Acoustics
Data modeling
Deconvolution
Network architectures
Signal generators
Image processing