|
ACCESS THE FULL ARTICLE
No SPIE Account? Create one
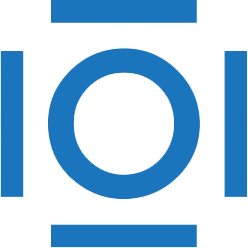
CITATIONS
Cited by 2 scholarly publications.
Image segmentation
Magnetic resonance imaging
Alzheimer's disease
Body temperature
Brain
Convolutional neural networks
Magnetism