|
ACCESS THE FULL ARTICLE
No SPIE Account? Create one
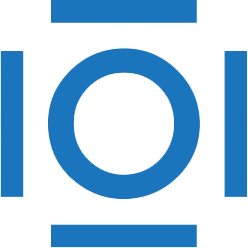
CITATIONS
Cited by 2 scholarly publications.
LIDAR
Sensors
Object recognition
Target recognition
Automatic target recognition
C++
Databases