|
ACCESS THE FULL ARTICLE
No SPIE Account? Create one
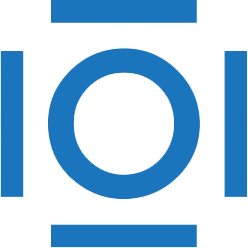
CITATIONS
Cited by 5 scholarly publications.
Medical imaging
Image analysis
Image filtering
Image segmentation
Pattern recognition
Tumors
Detection and tracking algorithms