|
ACCESS THE FULL ARTICLE
No SPIE Account? Create one
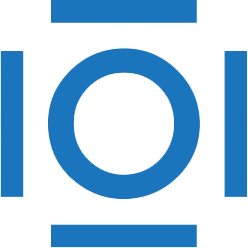
CITATIONS
Cited by 2 scholarly publications.
Radar
Quantization
Detection and tracking algorithms
Error analysis
Object recognition
Signal detection
Interference (communication)