|
ACCESS THE FULL ARTICLE
No SPIE Account? Create one
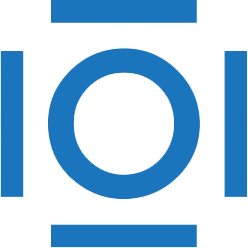
CITATIONS
Cited by 2 scholarly publications.
Neural networks
Signal processing
Manufacturing
Sensors
Surface roughness
Diagnostics
Network architectures