|
ACCESS THE FULL ARTICLE
No SPIE Account? Create one
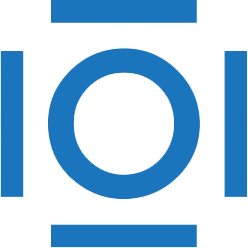
CITATIONS
Cited by 2 scholarly publications.
Wavelets
Convolution
Neural networks
Linear filtering
Pattern recognition
Wavelet transforms
Feature extraction