|
ACCESS THE FULL ARTICLE
No SPIE Account? Create one
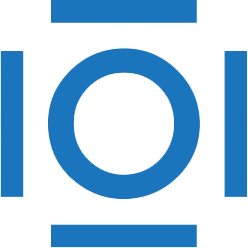
CITATIONS
Cited by 1 scholarly publication.
Machine learning
Network architectures
Artificial neural networks
Manufacturing
Neural networks
Binary data
Data modeling