|
ACCESS THE FULL ARTICLE
No SPIE Account? Create one
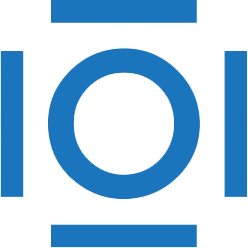
CITATIONS
Cited by 28 scholarly publications.
Principal component analysis
Feature extraction
Data analysis
Hyperspectral imaging
Data modeling
Image processing
Remote sensing