|
ACCESS THE FULL ARTICLE
No SPIE Account? Create one
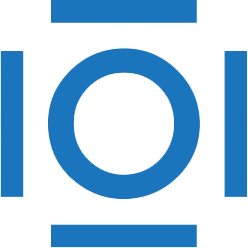
CITATIONS
Cited by 1 scholarly publication.
Image segmentation
Fuzzy logic
Image processing algorithms and systems
Lung
Information technology
Evolutionary algorithms
Image processing