|
ACCESS THE FULL ARTICLE
No SPIE Account? Create one
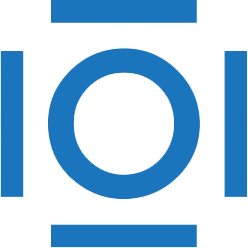
CITATIONS
Cited by 5 scholarly publications.
Binary data
Neural networks
Statistical analysis
Algorithm development
Neurons
Hyperspectral imaging
Network architectures