|
ACCESS THE FULL ARTICLE
No SPIE Account? Create one
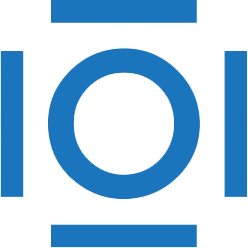
CITATIONS
Cited by 10 scholarly publications and 1 patent.
Sensors
Statistical modeling
Detection and tracking algorithms
Binary data
Performance modeling
Data modeling
Error analysis