|
ACCESS THE FULL ARTICLE
No SPIE Account? Create one
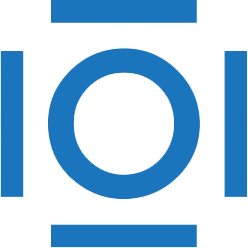
CITATIONS
Cited by 1 scholarly publication.
LIDAR
Clouds
Data modeling
Image classification
Image fusion
Remote sensing
Agriculture