|
ACCESS THE FULL ARTICLE
No SPIE Account? Create one
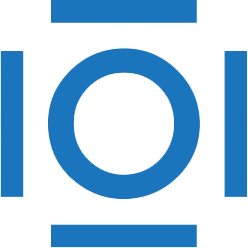
CITATIONS
Cited by 2 scholarly publications.
Data modeling
Autoregressive models
Sensors
Statistical modeling
Feature extraction
Error analysis
Statistical analysis