|
ACCESS THE FULL ARTICLE
No SPIE Account? Create one
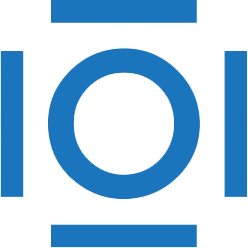
CITATIONS
Cited by 1 scholarly publication.
Data fusion
Detection and tracking algorithms
Reconstruction algorithms
Sensors
Pattern recognition
Algorithm development
Thulium