|
ACCESS THE FULL ARTICLE
No SPIE Account? Create one
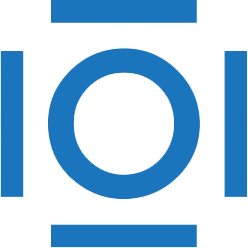
CITATIONS
Cited by 3 scholarly publications.
Composites
Mathematical modeling
Neural networks
Systems modeling
Data modeling
Algorithm development
Lithium