|
ACCESS THE FULL ARTICLE
No SPIE Account? Create one
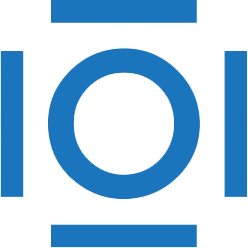
CITATIONS
Cited by 10 scholarly publications.
Error analysis
Associative arrays
Statistical analysis
Palladium
Detection theory
Signal to noise ratio
Statistical modeling