|
ACCESS THE FULL ARTICLE
No SPIE Account? Create one
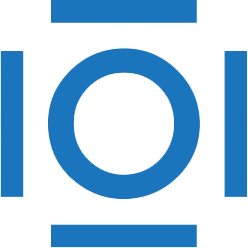
CITATIONS
Cited by 1 scholarly publication.
Detection and tracking algorithms
Image segmentation
Image processing
Object recognition
Signal processing
Evolutionary algorithms
Probability theory