|
ACCESS THE FULL ARTICLE
No SPIE Account? Create one
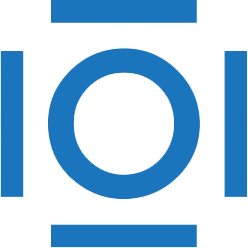
CITATIONS
Cited by 21 scholarly publications.
Synthetic aperture radar
Radar
Neural networks
Convolutional neural networks
Algorithm development
Machine learning
Automatic target recognition