We propose the convolutional neural networks approach for detecting urban slums from very high resolution (VHR) satellite images. Slums are becoming an inevitable and growing phenomenon in cities of the global south, whose locations are inappropriate in official statistics and maps. Thus the automatic detection and identification of slums provides vibrant information to decision-makers for formulating pro-poor policies in urban planning. However, field surveys are used as the conventional methods for slum detection, which are expensive and inefficient. The challenge is to find an automatic approach for identifying slums from VHR imagery. Although numerous studies focused on detecting slums from satellite data, only limited number captured their differences. Due to the unique spectral signatures of urban slums, it could not be simply classified as other urban (buildings, vegetation, and roads) features. We explore the potential of the dilated kernel-based deep convolutional neural network (DK-DCNN) approach to the learning of discriminatory spatial features and the automatic detection of slums from other features. However, the result obtained by the proposed DK-DCNN achieves high accuracy. The open area of informal settlements and the roofing structures of formal settlements are misclassified as slums. Morphological spatial pattern analysis, based on mathematical morphology, is used as a postprocessing method to enhance the classification accuracy. The four distinct very high-resolution satellite images captured by WorldView-2 Sensor (1.84 m) of Madurai and Tiruppur city, South India, have shown the performance of the proposed method to distinguish urban slums from other features by producing higher accuracy than any other approach. |
ACCESS THE FULL ARTICLE
No SPIE Account? Create one
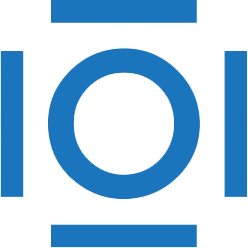
CITATIONS
Cited by 11 scholarly publications.
Convolution
Convolutional neural networks
Buildings
Image classification
Satellites
Accuracy assessment
Earth observing sensors