With the rapid development of optical remote sensing, it is urgent to find a reliable target detection method. Compared with traditional detection algorithms, a convolutional neural network has attracted considerable attention owing to its efficiency and high transitivity. However, different from general images, remote sensing images contain complex background information and dense small targets with changeable directions that make detection very challenging. To solve these problems and provide a stable and high-performance detection method, a rotated saliency fusion object detection (RSD) model based on “you only look once” (YOLO)v4 is established. First, salient image fusion technology is used to magnify target information. Second, the angle variable and rotated non-maximal suppression is introduced to improve the accuracy of rotated object detection by including the detection of dense objects. Third, the network structure is enhanced to improve the performance of small-target detection. Finally, the k-means algorithm and data enhancement are introduced to increase the robustness of the model. Extensive experiments demonstrate the superiority of the proposed model in detection speed and accuracy. The mean average precision of the proposed RSD model reaches 97.32% for the remote sensing images in a harbor area with an average detection speed of 13.41 s − 1. |
ACCESS THE FULL ARTICLE
No SPIE Account? Create one
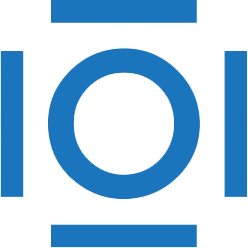
CITATIONS
Cited by 2 scholarly publications.
Target detection
Image fusion
Remote sensing
Detection and tracking algorithms
Performance modeling
Image segmentation
Data modeling