The Earth’s surface changes continuously due to several natural and humanmade factors. Efficient change detection (CD) is useful in monitoring and managing different situations. The recent rise in launched hyperspectral platforms provides a diversity of spectrum in addition to the spatial resolution required to meet recent civil applications requirements. Traditional multispectral CD algorithms hardly cope with the complex nature of hyperspectral images and their high dimensionality. To overcome these limitations, a CD deep convolutional neural network (CNN) semantic segmentation-based workflow was proposed. The proposed workflow is composed of four main stages, namely preprocessing, training, testing, and evaluation. Initially, preprocessing is performed to overcome hyperspectral image noise and the high dimensionality problem. Random oversampling (ROS), deep learning, and bagging ensemble were incorporated to handle imbalanced dataset. Also, we evaluated the generality and performance of the original UNet model and four variants of UNet, namely residual UNet, residual recurrent UNet, attention UNet, and attention residual recurrent UNet. Three hyperspectral CD datasets were employed in performance assessment for binary and multiclass change cases; all datasets suffer from class imbalance and small region of interest size. Recurrent residual UNet presented the best performance in both accuracy and inference time. Overall, the obtained results imply that deep CNN segmentation models can be utilized to implement efficient CD for hyperspectral imageries. |
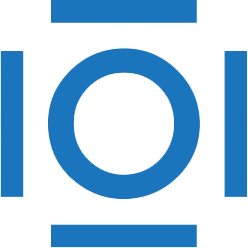
CITATIONS
Cited by 27 scholarly publications.
Image segmentation
Performance modeling
Neural networks
Hyperspectral imaging
Data modeling
Binary data
Process modeling