Significance: Biomedical optics system design, image formation, and image analysis have primarily been guided by classical physical modeling and signal processing methodologies. Recently, however, deep learning (DL) has become a major paradigm in computational modeling and has demonstrated utility in numerous scientific domains and various forms of data analysis. Aim: We aim to comprehensively review the use of DL applied to macroscopic diffuse optical imaging (DOI). Approach: First, we provide a layman introduction to DL. Then, the review summarizes current DL work in some of the most active areas of this field, including optical properties retrieval, fluorescence lifetime imaging, and diffuse optical tomography. Results: The advantages of using DL for DOI versus conventional inverse solvers cited in the literature reviewed herein are numerous. These include, among others, a decrease in analysis time (often by many orders of magnitude), increased quantitative reconstruction quality, robustness to noise, and the unique capability to learn complex end-to-end relationships. Conclusions: The heavily validated capability of DL’s use across a wide range of complex inverse solving methodologies has enormous potential to bring novel DOI modalities, otherwise deemed impractical for clinical translation, to the patient’s bedside. |
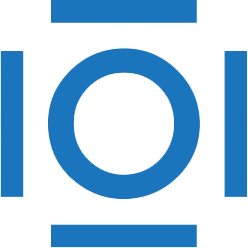
CITATIONS
Cited by 15 scholarly publications.
Diffuse optical imaging
Luminescence
Data modeling
Neural networks
Monte Carlo methods
Network architectures
Tissue optics