1.IntroductionCervical cancer is one of the leading causes of mortality in females worldwide.1,2 The number of incidences and mortality of cervical cancer is estimated to increase by 32% (from 604,127 to 797,712) and 41% (from 341,831 to 481,451) between 2020 and 2040, as reported by GLOBOCAN 2020 Report.1,3 Human papillomavirus (HPV) infection is the key cause of uncontrolled cell growth leading to cervical cancer. About 90% of cervical cancer is preventable because of the slow progression of the disease, giving a window of about 10 years for the precancerous lesion to be recognized and treated.4,5 The challenges for cervical cancer diagnosis are inter-observer variation in clinician’s interpretation, cost of cytology-based screening, and lack of skilled clinicians.6,7 The cervix is divided into the endocervical canal and ectocervix, lined by granular and squamous epithelium, respectively. The junction between the two epitheliums is known as the squamocolumnar junction (SCJ). In a person, the SCJ is originally present in the ectocervix, which then moves toward the endocervical canal during adolescence and pregnancy, forming the new SCJ. The region of epithelium between the old and the newly formed SCJ is called the transformation zone (T-zone), which is also prone to develop cervical lesions, as shown in Figs. 1(a) and 1(b).8 According to World Health Organization guidelines, a general screening for cervical cancer in women should be started ideally at the age 30 years followed by a regular screening at every 5 to 10 years. In HIV-positive women, the screening should be done at the age of 25 years followed by regular screening at every 3 to 5 years.5 Fig. 1(a) Uterus (frontal plane) with diseased cervix (yellow), (b) diseased cervix (transverse plane) with the lesion in yellow and the transformation zone represented by the gray shaded area. ![]() Conventional methods for detecting cervical cancer include direct methods, which are the gold standard techniques, such as the Pap-smear test and visual inspection with acetic acid and Lugol’s iodine.2,9,10 The Pap smear test involves the collection of cells from the transformation zone, which are stained and examined by clinicians to differentiate between normal and cancerous cells. The visual inspection, with or without colposcopy, involves assessing the cell’s nuclear morphology and reaction after applying acetic acid and Lugol’s iodine. The acetic acid applied on the surface of the cervical tissue denatures the protein and results in white patches, which can be observed visually. The growth, decay, and density of the white patch developed due to this coagulation of the proteins are used to delineate precancerous from normal. Lugol’s iodine quantifies the composition of the glycogen content, which is inversely proportional to the measure of cell division. The clinicians provide a Swede score based on five parameters: acetic acid uptake, margins, vessels, lesion size, and iodine uptake.11 If the Swede score exceeds five, the lesion is identified as a precancerous lesion requiring biopsy for pathological confirmation. However, acetic acid is corrosive in nature and causes discomfort to patients on application. Moreover, the variation in the clinician’s interpretation yields false positive and false negative results, leading to lower positive predictive value.12,13 Assays such as polymerase chain reaction (PCR) and next-generation sequencing (NGS) detect the HPV viral genome, suggesting the presence or absence of HPV infection may or may not be diagnostic for cervical cancer as most of the infections are self-limiting and disappear with time, and a prolong persistence of viral infection alone may lead to cervical precancer and cancer. Various conventional indirect methods have been used to detect HPV strains by polymerase chain reaction–restriction fragment length polymorphism based assay,14 NGS assay,15 and antigen capture by lateral flow assay; however, these molecular diagnostic techniques are time-consuming and require tedious sample preparation. Even though conventional diagnostic techniques have improved survival rates, there is a critical need for rapid and robust techniques that can be used in low-resource settings for accurate cervical cancer screening.16 Optical modality has been a key for cervical cancer diagnosis due to its cost-effectiveness, compactness, robustness, and reproducibility.17 Optical modalities have been used for breast cancer,18 skin cancer,19 oral cancer,20 gastrointestinal cancer,21 and bladder cancer.22 Several optical modalities have been implemented for cervical cancer diagnosis as well. Orfanoudaki et al. discussed the development of devices for better resolution images and the requirement for automated image analysis.23 Reviews carried out by Thekkek et al., Novikova et al, and Gonzalez et al. identified and described about the required development of portable, cost-effective optical devices for accurate diagnosis of the cervical cancer.24–26 This study highlights newer advancements in the development of probes and optical devices for point-of-care cervical cancer diagnosis at low-resource setting also combined with AI/ML for detailed image analysis. The key features of various optical techniques that have been developed for the detection of cervical precancer are highlighted, as shown in Fig. 2. 2.Optical Modalities for Cervical Cancer DetectionIn recent years, there has been significant progress in optical modalities, such as multispectral imaging, fluorescence imaging, polarization sensitive imaging, and Raman spectroscopy, and clinical procedures, such as colposcopy and endoscopy, which leverage optical technologies. In this section, different optical modalities based on white light imaging and fluorescence imaging for the detection of cervical precancer are discussed, and summarized in Table 1. Table 1Summary of the optical modalities for cervical cancer diagnosis.
EVA, enhanced visual assessment; HRME, high resolution microendoscopy; HPV, human papilloma virus; CIN, cervical intra-epithelial neoplasia; HSIL, high grade squamous intraepithelial lesion. 2.1.White Light ImagingWhite light imaging has been widely used to study cell and nuclear morphology. This modality provides a platform to visualize and study micro-sized cells and tissues to determine the underlying disease that affects cellular morphology and physiology. The visual inspection involves collecting the tissue biopsy, which is sliced in order of hundreds of micrometers and stained appropriately to assess the cancer biomarkers qualitatively. White light imaging techniques (visual inspection with acetic acid and visual inspection with Lugol’s iodine) also support the visual inspection with or without a colposcope. Here, we evaluate the degree of white patch intensity (due to acetic acid uptake) or yellow color intensity (due to Lugol’s iodine non-uptake) for initial cervical cancer screening. The recent development of white light imaging has shown the development of various types of transvaginal imaging probes for better visual inspection of the cervix. Asiedu et al. designed a user-friendly POCkeT colposcope that enables self-insertion and clinician-assisted image capturing of the cervix for cancer screening. The tool also enables easy application of acetic acid and Lugol’s iodine for further review.27 Aseidu et al. followed POCKeT with the developed machine learning (ML) algorithm that utilizes the images of acetic acid and Lugol’s iodine to treat the cervical tissues. The ML algorithm includes the selection of the region of interest of the cervix, reduction of specular reflectance, segmentation of selection region by Gabor algorithm, Haralick’s method for textural feature extraction, image processing based on different colors, and determination of the lesion size. Figures 3(a) and 3(c) show the image of the Pocket colposcope and the images captured by the system.28 Peterson et al. showed the application of a mobile-based colposcope with an enhanced visual assessment (EVA) system to support visual inspection by white light imaging for cancer screening.29 Acheampong et al. used a colposcope employed with an EVA system to detect cervical precancer in women while also assessing the prevalence of hr-HPV (high-risk human papillomavirus). The in-vivo images reported in the system are shown in Fig. 3(b), while the image processing steps are showcased in Fig. 3(c).30 A warm white LED-based white light source in a gynocular and colposcope device in a study performed by Nessa et al. for better detection of cervical lesions. The data collected by the devices were studied and analyzed by doctors to acquire information about acetowhiteness, lesion surface and size, glucose content, etc., to predict CIN 2+ (CIN 2, CIN 3, and invasive cervical cancer).31 Fig. 3(a) Schematic of cervix imaging using a Pocket colposcope.28 (b) Steps of image analysis of cervical lesions with the help of visual inspection by acetic acid and Lugol’s iodine.28 (Reprinted from Ref. 28 with permission of IEEE © 2018). (b) Cervix image of a patient who tested positive for HPV: (c.1) before acetic acid, (c.2) after acetic acid, (c.3) treatment-thermal coagulation.30 (Reprinted from Ref. 30 with permission of ecancer © 2021.) ![]() 2.2.Fluorescence ImagingThe fluorescence imaging modality consists of target-specific binding fluorophores and is used for obtaining qualitative and quantitative information about the desired target. The fluorescence imaging modality has higher sensitivity and resolution to detect cancer but is limited due to the lower intensity of fluorescence emitted by the fluorophores.55 The fluorescence imaging can be used as a label-free with endogenous fluorophores (NADH, flavins, porphyrins, and collagen) and label based with an exogenous fluorescent dye (ICG, fluorescein, rhodamine, and cyanine). Pierce et al. incorporated fluorescence imaging with a low-cost, high-resolution microendoscope (HRME). This technique required the use of an exogenous fluorescent dye, proflavine, that selectively stains cell nuclei and has an excitation and emission wavelength at 445 and 515 nm, respectively. HRME could identify the abnormality in the cervix by measuring the nuclear-cytoplasmic data in vivo and validating by biopsy studies of the same lesions.32 The capabilities of a fluorescence-based endomicroscopy to differentiate between normal columnar epithelium, stromal tissues, normal and precancerous squamous epithelium in vivo was explored and studied by Schlosser et al. In this study, acriflavine dye having an excitation wavelength of 445 nm was topically administered into the cervical region to measure the fluorescence emitted by the stained nuclei of the cells. The in-vivo images were then compared with the ex-vivo images of the biopsied cervical regions, which were then analyzed by stack analysis, as shown in Fig. 4(b). The authors reported that the device shown in Fig. 4(a) could assist in identifying subtle differences between normal and cancerous tissue types but cannot obtain and analyze data from deep tissues due to the lack of depth penetration of extrinsic fluorophores.33 Though fluorescence confocal microscopy cannot independently detect cervical cancer in vivo, promising results were shown in the diagnosis and screening of cervical cancer in ex-vivo conditions in a study by Sheikhzadeh et al. The diagnosis through the confocal images was validated using the histological images’ clinical interpretation. The instrument could obtain images at different depths of the cervical epithelium, such as below and analyze the images with a 92% to 100% sensitivity. This indicates that the fluorescence confocal microscopy can differentiate between normal cervical tissue, low-grade cervical intraepithelial neoplasia (CIN), and high-grade CIN in ex-vivo biopsy tissue based on features, such as nuclear area, cell density, estimated nuclear-to-cytoplasmic (ENC) ratio, and the average distance between a nucleus and its three nearest Delaunay neighbors.34 A study by Meena et al. detects and differentiates between normal and cancerous samples ex-vivo by measuring the fluorescence of FAD and elastic scattering of whole uterus samples using the system shown in Fig. 5(a). PCA and LDA analyzed the measured fluorescence and elastic scattering to differentiate between normal, CIN1, and CIN2. Figure 5(b) shows the difference in intrinsic fluorescence in normal, CIN1, and CIN2 lesions. This study raises significant interest in developing a similar probe for in-vivo detection and diagnosis of cervical cancer.35 Fig. 4In-vivo fluorescence endomicroscopy. (a) Image of the fluorescence-based endomicroscope. (b) Endomicroscopic images of normal squamous epithelium compared with the histological images. (c) Endomicroscopic images of precancerous squamous epithelium compared with histological images.33 (Reprinted from Ref. 33 with permission of SPIE © 2016.) ![]() Fig. 5(a)–(c) Intrinsic fluorescence-based portable device for cervical cancer detection. (a) Design of the instrument, (b) comparison of intrinsic fluorescence intensity of normal and CIN-1 lesions obtained from whole uterus samples, and (c) comparison of intrinsic fluorescence intensity of CIN1 and CIN2 lesions.35 (Reprinted from Ref. 35 with permission of SPIE © 2018.) ![]() Studies have been carried out to overcome the use of external fluorophores, also known as label-free fluorescence lifetime imaging (FLIM). The technique takes advantage of the autofluorescence property of NAD(P)H and FAD to obtain high-resolution images of the cervix region. Pouli et al. used label-free two-photon excited fluorescence technique to image the freshly excised biopsy samples for imaging and employed a laser of excitation wavelength 755 and 860 nm, and the emission is detected at and for NAD(P)H and FAD, respectively. The images are analyzed to observe the epithelial thickness, mitochondrial organization, and changes in the cellular metabolic changes as a marker to differentiate and classify between normal, low grade, and high grade squamous intraepithelial lesions (SIL).36 Ji et al. combined FLIM with ML algorithms for imaging of exfoliated cervical cell samples that helps in predicting risk of cervical cancer in the patients with a sensitivity and specificity of 90.9% and 100%, respectively.37 Wang et al. used phasor analysis for the image analysis obtained from FLIM that helps in distinguishing normal, low, and, high grade cervical lesions based on the relationship between metabolic changes and cancer development.38 Qiu et al. performed a strong near-infrared absorption based fluorescence imaging for the early diagnosis of cervical cancer.56 2.3.Raman SpectroscopyRaman spectroscopy has been extensively explored for the early detection of cancers. In the study by Shaikh et al., the authors studied the ability of Raman spectroscopy to detect cervical cancer. The study revealed that the Raman spectroscopy was a better method for non-invasive cancer at low-resource settings by identifying peak intensity differences for hemoglobin and non-collagenous proteins between tumorous cervical tissues and normal tissues, as shown in Figs. 6(a)–6(c).39 Raman spectroscopy for in-vivo detection of cervical cancer based on peak differences at 1006, 1058, 1086, 1244, 1270, 1324, 1450, 1550, and has been used to classify high-grade dysplasia, low-grade dysplasia, squamous metaplasia, and normal ectocervix using multiclass diagnostic algorithms, respectively.40 Cervical cancer has also been differentiated from normal samples by using near-infrared (NIR) based Raman spectroscopy in the study performed by Raja et al. In this study, blood samples of patients diagnosed with cervical cancer and normal patients were used to collect Raman signals. The signals from the normal and cancerous blood samples were compared, indicating that the peak intensity of some amino acids, such as phenylalanine, tryptophan, and tyrosine, and nucleic acids, such as adenine, increases in the case of cancer, a basis for considering as cancer biomarkers.41 Zhang et al., in their study, took formalin-fixed cervical tissue samples collected from patients diagnosed with cervical adenocarcinoma and cervical squamous cell carcinoma. These samples were used to collect the Raman spectrum, which was compared and analyzed using multiple ML algorithms, such as adaptive iteratively reweighted penalized least squares (airPLS) algorithm, Vancouver Raman algorithm, partial least squares (PLS), principal component analysis (PCA), kernel PCA, isometric feature mapping (isomap), and locally linear embedding. The algorithms were used to identify quantitative and qualitative differences between the two Raman spectrums and classify the samples into the two types of cancers. Out of all the algorithms, airPLS-PLS-KNN shows the highest accuracy of 96% for screening cancer.42 A recent study by Yang et al. uses Raman spectroscopy to identify and differentiate various cervical tissues and related diseases. In the study, the authors developed a confocal Raman spectrometer with a laser excitation of 532 nm to record the spectrum of cervical tissue samples collected from patients suffering from cervicitis, low-grade and high-grade SIL, cervical squamous cell carcinoma, and cervical adenocarcinoma. The conditions mentioned above were differentiated based on the spectral differences of proteins, carbohydrates, and nucleic acids, as shown in Fig. 6(d), which was further analyzed and differentiated using linear and non-linear classification models.43 Fig. 6(a) Cancerous site of cervix, (b) normal cervical tissues, and (c) normal vaginal sites.39 (Reprinted from Ref. 39 with permission of Wiley © 2017.) (d) Spectral differences between cervicitis, LSIL, HSIL, cervical squamous cell carcinoma, and cervical adenocarcinoma.43 (Reprinted from Ref. 43 with permission of Wiley © 2021.) (LSIL, low-grade squamous intraepithelial lesion; HSIL, high-grade squamous intraepithelial lesion.) ![]() 2.4.Polarization-Sensitive SpectroscopyMueller matrix polarimeters have been recently studied for their ability to accurately diagnose cancer. The imaging system is highly sensitive and accurate, has high measurement speed, and can perform multicomponent analysis.44 Ramella-Roman et al. developed a snapshot Mueller matrix polarimeter that measures the polarization of collagen fibers in the cervix with the help of an LED operating at 633 nm was developed. The study reveals that any deviation from the standard polarization of collagen can indicate a diseased condition.45 Kupinski et al. developed a polarization-sensitive imaging technique while operating at 450 to 700 nm and acquiring 16 images at each wavelength to reconstruct the Mueller matrix of the sample. Radiance images acquired at 550 nm showed a low signal-to-noise ratio with high sensitivity and specificity. Figure 7 shows the images of 24 excised cervical samples in accordance with histopathological studies.46 Zaffar et al. describe that polarization dynamics can be studied to differentiate between epithelium and stroma of cervical tissue. The study further explains the differentiation between the two types of tissue based on the picosecond result intensity patterns at different time delays recorded with the Mueller matrix polarimeter.47 Zaffar et al., in the follow-up study, described that the orientations of collagen fibers randomize during disease progression. The orientations have been acquired in the form of matrix images, which have been analyzed using the Fourier domain. Based on the first row and column elements, the Fourier spectrum differentiates between cervical neoplasia (CIN-1, CIN-2) from the normal tissues.48 Liu et al. described the use of a polarization-based multi-parametric imaging system compared with conventional microscopy’s ability to resolve fine structural features of the nucleus in the cervical cells. This study sheds light on the application of polarization multi-parametric imaging to diagnose cervical precancers based on Pap-smear test and nuclear-cytoplasmic density.49 Roa et al. described the identification and classification of collagen and elastin fibers in cervical tissues based on the microscopic images acquired by Mueller matrix polarimeter combined with convolutional neural networks and -nearest neighbor for classification. The study was performed on mice to observe and classify the different tissue fibers.57 Such studies prove the application of polarization-sensitive imaging to detect and diagnose cervical cancer based on different structural features, such as the orientation of the fibers and resulting anisotropy. 2.5.Narrow-Band ImagingNarrow band imaging enables clear and high-resolution visualization of tissue structures, allowing a precise and enhanced diagnosis of lesions. The earliest work on the use of multispectral imaging for cervical cancer diagnosis was performed by Benavides et al. while identifying the critical operating wavelength range from 330 and 360 nm and 440 to 470 nm.58 Yi et al. developed a computer-aided multispectral narrow-band imaging (NBI) system, as shown in Fig. 8(a), operating at four wavelengths 415, 450, 525, and 620 nm to capture multiple images of the cervix at zero-lag time. The images were then fused and processed using the Euclidean distance classification algorithm to classify normal and cancerous tissues, as shown in Fig. 8(b). The main advantage of this technique is that it does not require external reagents to stain the cervix and diagnose cancer.50 Kobara et al. compared NBI with colposcopy in detecting CIN and CIN2+ lesions. The study showed that NBI is highly sensitive (87%) than colposcopy (74%) but has a low specificity (50%) when compared with that of colposcopy (68%). It also describes that NBI performs similarly to colposcopy while detecting CIN2+ lesions. Figure 8(c) shows the system’s image, and Fig. 8(d) shows the difference between a colposcopic image and NBI image.51 Uchita et al. described cervical cancer screening by combining the magnifying endoscopy and narrow-band imaging (ME-NBI). The differentiation between CIN and non-CIN tissues was made based on the presence of light white epithelium and heavy white epithelium with atypical intra-epithelial papillary capillary loop. The ME-NBI system enabled the clear visualization of tissue structures.52 Fig. 8(a) Computer-aided narrow-band snapshot MSI system, (b) fused image of NBI snapshot processed with Euclidean classification algorithm in which green, gray, pink, and red regions indicate normal, CIN2, CIN3, carcinoma in situ, respectively.50 (Reprinted from Ref. 50 with permission of Springer © 2020.) (c) ME-NBI system, (d) NBI imaging showing thin white epithelium (yellow box) and CIN1 confirmed by biopsy (red box).51 (Reprinted from Ref. 51 with permission of MDPI © 2021.) ![]() 2.6.Multimodal TechniquesAlthough single-modal imaging systems and detection probes provide significant information to the clinician for the diagnosis of cervical cancer, the multimodal approach helps in further validation and confirmation of the data obtained for higher accuracy in diagnosing cervical cancer. Coole et al. reported a multimodal imaging system for detecting cervical precancerous lesions. The system consists of a POCket colposcope integrated with HRME, which brings together the ability to measure the acetowhiteness of the lesions and fluorescence from the nuclei. The system employed an illuminator of a wavelength of 460 nm to excite the fluoropore proflavine dye that stains the nuclei. The images obtained, as shown in Figs. 9(a)–9(d), are analyzed by multi-task neural network that studied the nuclear characteristics and classified high-grade and low-grade cervical precancer or cancer.53 Gordon et al. detected SIL in the cervix region at an early stage by combining three different modalities into a probe system. The Micro Colposcope Probe detects SIL by combining white light reflected spectroscopy, coherent light scattering, and high-magnification, high-resolution RGB imaging. The probe consisted of four LEDs, a monochromatic infrared laser, and an RGB camera. Positive samples differentiated the negative samples by observing flat spectral signals, dark, and less textured scattering images, and patterns of blood vessels.54 3.AI Approaches for Cervical Cancer diagnosisArtificial intelligence (AI) and ML techniques have shown great potential in the field of medical image analysis, including the detection and diagnosis of cervical cancer. Recent advances in AI/ML algorithms have led to significant improvements in accuracy, speed, and efficiency in cervical cancer diagnosis. AI/ML algorithms can analyze colposcopy images and provide an accurate diagnosis, reducing the need for unnecessary biopsies. Jayabk et al. utilized image registration, feature extraction, and morphological operations to identify cancerous and non-cancerous cervical images with an accuracy of 97.14% and 100%, respectively. The adaptive neuro-fuzzy inference system was used as the method for classification.59 Kim et al. used filtering, preprocessing, and normalization techniques to extract AI features for cervical cancer detection. The authors developed the Cerviray AI and achieved an overall accuracy of 72% with a specificity of 83.05%.60 Wong et al. used hr-HPV testing, testing and training data, and classification methods, such as decision tree, random forest (RF), and SVM to classify CIN1, CIN2, CIN3, and CIN3+ with an accuracy of 96.88% and specificity of 94.32%.61 Tian et al. focused on the identification of HPV genotypes and somatic mutations for the diagnosis of cervical cancer. The authors utilized an RF unsupervised cluster to identify 17 types of HPV with an accuracy of 81.4%.62 Mehmood et al. applied data cleaning and feature selection techniques using the RF algorithm, forward propagation, backward propagation, and shallow neural network to diagnose cervical cancer. The algorithm used the Pearson correlation method to identify the most significant features for classification.63 Overall, these studies demonstrate the potential of AI/ML in the accurate and timely diagnosis of cervical cancer. The various techniques and methods employed in these studies can be combined and expanded to develop more robust and effective cervical cancer diagnosis tools. These approaches have been tabulated in Table 2. Table 2Summary of various ML approaches toward cervical cancer diagnosis.
4.ConclusionThe studies described in this article individually have significant potential to be used as a screening technique for cervical cancer. However, there are also disadvantages, such as invasiveness, reproducibility of imaging system, interpatient variation, time-consuming sample preparation, variation in clinician’s interpretation, trained clinicians, and data scientists for image processing and diagnosis. While the analysis can be made simpler using AI where the ML algorithms could process the images and predict the disease as a normal, low-grade lesion or high-grade lesion with high accuracy. In such scenario, a cervical screening probe can be used as a point-of-care testing device. Raman spectroscopy, combined with other techniques, serves as another alternative to multimodal imaging systems due to its ability to detect any tissue changes based on the spectra. Data analysis for Raman spectroscopy can be better supported with ML algorithms and databases. Apart from these, multimodal systems overcome the disadvantages and limitations of the individual systems by each system supporting the other system. The multimodal system combining the individual sensitivity of two or more techniques gives an accumulated higher accuracy to differentiate between a normal tissue and a precancerous lesion and a confirmed diagnosis of the disease, thereby reducing false positives and negative results. The AI/ML techniques have shown great potential in improving the accuracy and efficiency of a cervical cancer diagnosis. However, further research is required to validate these techniques in large-scale clinical trials and integrate them into routine clinical practice. With continued research and development, AI/ML along with multimodal optical approaches can significantly reduce the burden of cervical cancer worldwide. ReferencesS. Zhang et al.,
“Cervical cancer: epidemiology, risk factors and screening,”
Chin. J. Cancer Res., 32
(6), 720 https://doi.org/10.21147/j.issn.1000-9604.2020.06.05
(2020).
Google Scholar
World Health Organization, World Fact Sheet, International Agency for Research on Cancer(
(2020). https://gco.iarc.fr/today/data/factsheets/populations/900-world-fact-sheets.pdf Google Scholar
J. Ferlay et al., Global and regional estimates of the incidence and mortality for 38 cancers: GLOBOCAN 2018, World Health Organization, International Agency for Research on Cancer, Lyon, France
(2018). Google Scholar
M. A. Vink et al.,
“Primary human papillomavirus DNA screening for cervical cancer prevention: can the screening interval be safely extended?,”
Int. J. Cancer, 137
(2), 420
–427 https://doi.org/10.1002/ijc.29381 IJCNAW 1097-0215
(2015).
Google Scholar
World Health Organization, United Nations Population Fund,
“Preparing for the introduction of HPV vaccines: policy and programme guidance for countries,”
(2006). Google Scholar
A. Adedimeji et al.,
“Challenges and opportunities associated with cervical cancer screening programs in a low income, high HIV prevalence context,”
BMC Women’s Health, 21
(1), 74 https://doi.org/10.1186/s12905-021-01211-w
(2021).
Google Scholar
H. A. Cubie and C. Campbell,
“Cervical cancer screening: the challenges of complete pathways of care in low-income countries: focus on Malawi,”
Women’s Health, 16 1745506520914804 https://doi.org/10.1177/1745506520914804
(2020).
Google Scholar
W. Prendiville and R. Sankaranarayanan, Colposcopy and Treatment of Cervical Pre-Cancer, International Agency for Research on Cancer, World Health Organization(
(2017). Google Scholar
B. K. Desire et al.,
“Visual inspection with acetic acid and Lugol’s iodine in cervical cancer screening at the general referral hospital Kayembe in Mbuji-Mayi, Democratic Republic of Congo,”
Pan Afr. Med. J., 23
(1), 64 https://doi.org/10.11604/pamj.2016.23.64.8450
(2016).
Google Scholar
P. Ghosh et al.,
“Visual inspection of cervix with Lugol’s iodine for early detection of premalignant & malignant lesions of cervix,”
Indian J. Med. Res., 136
(2), 265
(2012).
Google Scholar
J. Bowring et al.,
“The Swede score: evaluation of a scoring system designed to improve the predictive value of colposcopy,”
J. Lower Genital Tract Dis., 14
(4), 301
–305 https://doi.org/10.1097/LGT.0b013e3181d77756
(2012).
Google Scholar
D. R. Grimes et al.,
“Challenges of false positive and negative results in cervical cancer screening,”
(2020). Google Scholar
P. Dalla Palma et al.,
“The risk of false-positive histology according to the reason for colposcopy referral in cervical cancer screening: a blind revision of all histologic lesions found in the NTCC trial,”
Am. J. Clin. Pathol., 129
(1), 75
–80 https://doi.org/10.1309/EWYGWFRRM8798U5P AJCPAI 0002-9173
(2008).
Google Scholar
I. M. A. Melo et al.,
“PCR-RFLP and FTIR-based detection of high-risk human papilloma virus for cervical cancer screening and prevention,”
Biochem. Biophys. Rep., 26 100993 https://doi.org/10.1016/j.bbrep.2021.100993
(2021).
Google Scholar
X. Sastre-Garau et al.,
“A NGS-based blood test for the diagnosis of invasive HPV-associated carcinomas with extensive viral genomic characterization,”
Clin. Cancer Res., 27
(19), 5307
–5316 https://doi.org/10.1158/1078-0432.CCR-21-0293
(2021).
Google Scholar
K. A. Kundrod et al.,
“Advances in technologies for cervical cancer detection in low-resource settings,”
Expert Rev. Mol. Diagn., 19
(8), 695
–714 https://doi.org/10.1080/14737159.2019.1648213
(2019).
Google Scholar
U. M. Pal et al.,
“Optical spectroscopy-based imaging techniques for the diagnosis of breast cancer: a novel approach,”
Appl. Spectrosc. Rev., 55
(8), 778
–804 https://doi.org/10.1080/05704928.2020.1749651 APSRBB 0570-4928
(2020).
Google Scholar
U. M. Pal et al.,
“Hybrid spectral-IRDx: near-IR and ultrasound attenuation system for differentiating breast cancer from adjacent normal tissue,”
IEEE Trans. Biomed. Eng., 68
(12), 3554
–3563 https://doi.org/10.1109/TBME.2021.3077582 IEBEAX 0018-9294
(2021).
Google Scholar
L. Rey-Barroso et al.,
“Optical technologies for the improvement of skin cancer diagnosis: a review,”
Sensors, 21
(1), 252 https://doi.org/10.3390/s21010252 SNSRES 0746-9462
(2021).
Google Scholar
N. Subhash et al.,
“Bimodal multispectral imaging system with cloud-based machine learning algorithm for real-time screening and detection of oral potentially malignant lesions and biopsy guidance,”
J. Biomed. Opt., 26
(8), 086003 https://doi.org/10.1117/1.JBO.26.8.086003 JBOPFO 1083-3668
(2021).
Google Scholar
Y. Tang, S. Anandasabapathy and R. Richards-Kortum,
“Advances in optical gastrointestinal endoscopy: a technical review,”
Mol. Oncol., 15
(10), 2580
–2599 https://doi.org/10.1002/1878-0261.12792
(2021).
Google Scholar
J. J. Liu, M. J. Droller and J. C. Liao,
“New optical imaging technologies for bladder cancer: considerations and perspectives,”
J. Urol., 188
(2), 361
–368 https://doi.org/10.1016/j.juro.2012.03.127
(2012).
Google Scholar
I. M. Orfanoudaki, D. Kappou and S. Sifakis,
“Recent advances in optical imaging for cervical cancer detection,”
Arch. Gynecol. Obstet., 284
(5), 1197
–1208 https://doi.org/10.1007/s00404-011-2009-4
(2011).
Google Scholar
N. Thekkek and R. Richards-Kortum,
“Optical imaging for cervical cancer detection: solutions for a continuing global problem,”
Nat. Rev. Cancer, 8
(9), 725
–731 https://doi.org/10.1038/nrc2462 NRCAC4 1474-175X
(2008).
Google Scholar
T. Novikova,
“Optical techniques for cervical neoplasia detection,”
Beilstein J. Nanotechnol., 8
(1), 1844
–1862 https://doi.org/10.3762/bjnano.8.186 BJNEAH 2190-4286
(2017).
Google Scholar
M. Gonzalez et al.,
“Cervical imaging in the low resource setting: a review,”
Biosensors, 12
(10), 786 https://doi.org/10.3390/bios12100786 BISSED 0265-928X
(2022).
Google Scholar
M. N. Asiedu et al.,
“Design and preliminary analysis of a vaginal inserter for speculum-free cervical cancer screening,”
PLoS One, 12
(5), e0177782 https://doi.org/10.1371/journal.pone.0177782 POLNCL 1932-6203
(2017).
Google Scholar
M. N. Asiedu et al.,
“Development of algorithms for automated detection of cervical pre-cancers with a low-cost, point-of-care, pocket colposcope,”
IEEE Trans. Biomed. Eng., 66
(8), 2306
–2318 https://doi.org/10.1109/TBME.2018.2887208 IEBEAX 0018-9294
(2018).
Google Scholar
C. W. Peterson et al.,
“Real-time monitoring and evaluation of a visual-based cervical cancer screening program using a decision support job aid,”
Diagnostics, 6
(2), 20 https://doi.org/10.3390/diagnostics6020020
(2016).
Google Scholar
L. K. Acheampong et al.,
“Determining the prevalence of high-risk human papillomavirus infection using a novel cervical pre-cancer screening approach in incarcerated women at the Nsawam Medium Security Prison, Ghana,”
Ecancermedicalscience, 15 1248 https://doi.org/10.3332/ecancer.2021.1248
(2021).
Google Scholar
A. Nessa et al.,
“Evaluation of the accuracy in detecting cervical lesions by nurses versus doctors using a stationary colposcope and gynocular in a low-resource setting,”
BMJ Open, 4
(11), e005313 https://doi.org/10.1136/bmjopen-2014-005313
(2014).
Google Scholar
M. C. Pierce et al.,
“A pilot study of low-cost, high-resolution microendoscopy as a tool for identifying women with cervical precanceroptical imaging for detection of cervical pre-cancer,”
Cancer Prevent. Res., 5
(11), 1273
–1279 https://doi.org/10.1158/1940-6207.CAPR-12-0221
(2012).
Google Scholar
C. L. Schlosser et al.,
“Fluorescence confocal endomicroscopy of the cervix: pilot study on the potential and limitations for clinical implementation,”
J. Biomed. Opt., 21
(12), 126011 https://doi.org/10.1117/1.JBO.21.12.126011 JBOPFO 1083-3668
(2016).
Google Scholar
F. Sheikhzadeh et al.,
“Quantification of confocal fluorescence microscopy for the detection of cervical intraepithelial neoplasia,”
BioMed. Eng. OnLine, 14
(1), 96 https://doi.org/10.1186/s12938-015-0093-6
(2015).
Google Scholar
B. L. Meena et al.,
“Intrinsic fluorescence for cervical pre-cancer detection using polarized light based in-house fabricated portable device,”
J. Biomed. Opt., 23
(1), 015005 https://doi.org/10.1117/1.JBO.23.1.015005 JBOPFO 1083-3668
(2018).
Google Scholar
D. Pouli et al.,
“Label-free, high-resolution optical metabolic imaging of human cervical precancers reveals potential for intraepithelial neoplasia diagnosis,”
Cell Rep. Med., 1
(2), 100017 https://doi.org/10.1016/j.xcrm.2020.100017
(2020).
Google Scholar
M. Ji et al.,
“Early detection of cervical cancer by fluorescence lifetime imaging microscopy combined with unsupervised machine learning,”
Int. J. Mol. Sci., 23
(19), 11476 https://doi.org/10.3390/ijms231911476 1422-0067
(2022).
Google Scholar
X. Wang et al.,
“Discriminating different grades of cervical intraepithelial neoplasia based on label-free phasor fluorescence lifetime imaging microscopy,”
Biomed. Opt. Express, 11
(4), 1977
–1990 https://doi.org/10.1364/BOE.386999 BOEICL 2156-7085
(2020).
Google Scholar
R. Shaikh et al.,
“A comparative evaluation of diffuse reflectance and Raman spectroscopy in the detection of cervical cancer,”
J. Biophotonics, 10
(2), 242
–252 https://doi.org/10.1002/jbio.201500248
(2017).
Google Scholar
E. M. Kanter et al.,
“Multiclass discrimination of cervical pre-cancers using Raman spectroscopy,”
J. Raman Spectrosc., 40
(2), 205
–211 https://doi.org/10.1002/jrs.2108 JRSPAF 0377-0486
(2017).
Google Scholar
P. Raja et al.,
“Characterization of blood plasma of normal and cervical cancer patients using NIR Raman spectroscopy,”
Vib. Spectrosc., 102 1
–7 https://doi.org/10.1016/j.vibspec.2019.03.001 VISPEK 0924-2031
(2019).
Google Scholar
H. Zhang et al.,
“Rapid identification of cervical adenocarcinoma and cervical squamous cell carcinoma tissue based on Raman spectroscopy combined with multiple machine learning algorithms,”
Photodiagn. Photodyn. Ther., 33 102104 https://doi.org/10.1016/j.pdpdt.2020.102104
(2021).
Google Scholar
B. Yang et al.,
“Feature fusion combined with tissue Raman spectroscopy to screen cervical cancer,”
J. Raman Spectrosc., 52
(11), 1830
–1837 https://doi.org/10.1002/jrs.6246 JRSPAF 0377-0486
(2021).
Google Scholar
D. N. Ignatenko et al.,
“Applications of Mueller matrix polarimetry to biological and agricultural diagnostics: a review,”
Appl. Sci., 12
(10), 5258 https://doi.org/10.3390/app12105258
(2022).
Google Scholar
J. C. Ramella-Roman et al.,
“Development and characterization of a snapshot Mueller matrix polarimeter for the determination of cervical cancer risk in the low resource setting,”
Proc. SPIE, 10485 109
–120 https://doi.org/10.1117/12.2290592 PSISDG 0277-786X
(2018).
Google Scholar
M. Kupinski et al.,
“Polarimetric measurement utility for pre-cancer detection from uterine cervix specimens,”
Biomed. Opt. Express, 9
(11), 5691
–5702 https://doi.org/10.1364/BOE.9.005691 BOEICL 2156-7085
(2018).
Google Scholar
M. Zaffar, G. R. Sahoo and A. Pradhan,
“Different orders of scattering through time-resolved Mueller matrix imaging estimates of pre-malignancy in human cervical tissues,”
Appl. Opt., 59
(14), 4286
–4295 https://doi.org/10.1364/AO.389786 APOPAI 0003-6935
(2020).
Google Scholar
M. Zaffar and A. Pradhan,
“Assessment of anisotropy of collagen structures through spatial frequencies of Mueller matrix images for cervical pre-cancer detection,”
Appl. Opt., 59
(4), 1237
–1248 https://doi.org/10.1364/AO.377105 APOPAI 0003-6935
(2020).
Google Scholar
W. Liu et al.,
“Polarization multi-parametric imaging method for the inspection of cervix cell,”
Opt. Commun., 488 126846 https://doi.org/10.1016/j.optcom.2021.126846 OPCOB8 0030-4018
(2021).
Google Scholar
D. Yi, L. Kong and Y. Zhao,
“Contrast-enhancing snapshot narrow-band imaging method for real-time computer-aided cervical cancer screening,”
J. Digit. Imaging, 33 211
–220 https://doi.org/10.1007/s10278-019-00215-1 JDIMEW
(2020).
Google Scholar
H. Kobara et al.,
“Flexible magnifying endoscopy with narrow band imaging for diagnosing uterine cervical neoplasms: a multicenter prospective study,”
J. Clin. Med., 10
(20), 4753 https://doi.org/10.3390/jcm10204753
(2021).
Google Scholar
K. Uchita et al.,
“Characteristic findings of high-grade cervical intraepithelial neoplasia or more on magnifying endoscopy with narrow band imaging,”
Int. J. Clin. Oncol., 23 707
–714 https://doi.org/10.1007/s10147-018-1247-x
(2018).
Google Scholar
J. B. Coole et al.,
“Development of a multimodal mobile colposcope for real-time cervical cancer detection,”
Biomed. Opt. Express, 13
(10), 5116
–5130 https://doi.org/10.1364/BOE.463253 BOEICL 2156-7085
(2022).
Google Scholar
S. Gordon et al.,
“A novel multimodal optical imaging device for cervical cancer screening and diagnosis,”
Proc. SPIE, 10856 38
–46 https://doi.org/10.1117/12.2508338 PSISDG 0277-786X
(2019).
Google Scholar
T. Wang et al.,
“Recent progress in second near-infrared (NIR-II) fluorescence imaging in cancer,”
Biomolecules, 12
(8), 1044 https://doi.org/10.3390/biom12081044
(2022).
Google Scholar
T. Qiu et al.,
“In vivo multi-scale photoacoustic imaging guided photothermal therapy of cervical cancer based on customized laser system and targeted nanoparticles,”
Int. J. Nanomed., 16 2879
–2896 https://doi.org/10.2147/IJN.S301664
(2021).
Google Scholar
C. Roa et al.,
“Auto-detection of cervical collagen and elastin in Mueller matrix polarimetry microscopic images using K-NN and semantic segmentation classification,”
Biomed. Opt. Express, 12
(4), 2236
–2249 https://doi.org/10.1364/BOE.420079 BOEICL 2156-7085
(2021).
Google Scholar
J. M. Benavides et al.,
“Multispectral digital colposcopy for in vivo detection of cervical cancer,”
Opt. Express, 11
(10), 1223
–1236 https://doi.org/10.1364/OE.11.001223 OPEXFF 1094-4087
(2003).
Google Scholar
B. K. Jaya and S. S. Kumar,
“Image registration based cervical cancer detection and segmentation using ANFIS classifier,”
Asian Pac. J. Cancer Prevent., 19
(11), 3203 https://doi.org/10.31557/APJCP.2018.19.11.3203
(2018).
Google Scholar
S. Kim et al.,
“Role of artificial intelligence interpretation of colposcopic images in cervical cancer screening,”
Healthcare, 10
(3), 468 https://doi.org/10.3390/healthcare10030468
(2022).
Google Scholar
O. G. Wong et al.,
“Machine learning interpretation of extended human papillomavirus genotyping by onclarity in an Asian cervical cancer screening population,”
J. Clin. Microbiol., 57
(12), e00997
–19 https://doi.org/10.1128/JCM.00997-19 JCMIDW 1070-633X
(2019).
Google Scholar
R. Tian et al.,
“Risk stratification of cervical lesions using capture sequencing and machine learning method based on HPV and human integrated genomic profiles,”
Carcinogenesis, 40
(10), 1220
–1228 https://doi.org/10.1093/carcin/bgz094 CRNGDP 1460-2180
(2019).
Google Scholar
M. Mehmood et al.,
“Machine learning assisted cervical cancer detection,”
Front. Public Health, 9 788376 https://doi.org/10.3389/fpubh.2021.788376
(2021).
Google Scholar
BiographyAlisha Rahaman was pursuing her Master of Science degree in the Department in Microbiology at the Savitribai Phule Pune University while working on this review paper. Her field of interest is disease diagnosis using various spectroscopy modalities such as Raman spectroscopy. Arpitha Anantharaju is an associate professor, Department of Obstetrics and Gynaecology, JIPMER. She has expertise in the qualitative aspects of management of oncological patients, cytoreductive surgeries, preventive, and palliative care. Karthika Jeyachandran is currently pursuing her PhD in biomedical engineering at the Department of Sciences and Humanities, IIITDM Kancheepuram-Chennai. Her primary focus lies in the field of optical imaging, specifically in the domain of medical diagnosis. Through her research, she strives to explore and harness the potential of optical imaging techniques for enhanced and more accurate medical diagnostics. Her dedication and commitment to advancing this field highlight her passion for improving healthcare outcomes through innovative imaging technologies. Repala Manideep is currently pursuing his Bachelor of Technology degree in engineering in the Department of Electronic and Communication Engineering, IIITDM Kancheepuram-Chennai. His field of interest is in machine learning and artificial intelligence. Uttam M. Pal is an assistant professor in the Department of Electronic and Communication Engineering, IIITDM Kancheepuram-Chennai. His research focus is interdisciplinary in nature to address the challenges in biomedical diagnostic devices, including microscopic and macroscopic investigations. He has expertise in developing novel optical tools for intraoperative cancer margin assessment and cancer diagnosis. |
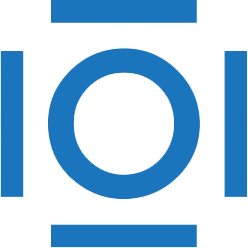
CITATIONS
Cited by 1 scholarly publication.
Cervical cancer
Cancer detection
Tissues
Cancer
Raman spectroscopy
Optical imaging
Cervix