1.IntroductionHyperspectral imaging is imaging of a target at a large number of discrete wavelengths. In its simplest form, a hyperspectral image forms a “data cube” in which two dimensions represent distances in space, while the third dimension represents spectral wavelength or wave number. Additional dimensions for time and even inter-wavelength correlations are also possible. Hyperspectral imaging is typically used in aerospace and defense applications, but applications in pharmaceutical analysis are also feasible.1 Technology is available for hyperspectral imaging at very long distances. The Predator remotely piloted reconnaissance aircraft is capable of hyperspectral imaging in the visible and infrared regions.2 The NASA near earth asteroid rendezvous spacecraft launched in 1996 began to orbit the asteroid Eros in 2000 at a distance of 200 km, and collected spectra from 804–2732 nm.3 These spectra revealed that Eros is a moderately porous, chondritic asteroid with a composition similar to that of the protoplanetary disk. These successes suggest that a portable hyperspectral-imaging device might even be useful in detecting contamination in the changeover between production of different pharmaceutical materials. The perfect method for validation of cleaning would be capable of determining multiple chemical constituents and their locations simultaneously. The method would be quick and easy to use. Measurements made with the technique would be free of systematic bias and would be highly reproducible. The method would have good dynamic range and would be selective, so no characteristic of the environment would interfere with the measurement of any analyte. As the instrument approaches the ideal “analytical black box,” it would be able to recognize that it is examining a sample unlike any it has ever examined before and would respond appropriately. This response could take the form of a request for operator assistance or for more samples of the same type, a “second opinion” analysis by another technique, or a library search for the best step to take. Hyperspectral imaging and chemometrics are increasingly approaching this ideal. The purpose of this research was to lay the groundwork for cleaning validation experiments by: 1. providing an indication of how much distance can be between near-infrared (IR) instrumentation and the sample while still maintaining adequate signal-to-noise ratio (S/N) in a single-capsule analysis, and 2. providing an indication of spatial resolution and how many samples can be analyzed simultaneously while maintaining an adequate S/N and pixel coverage on each tablet in a multiple-tablet analysis. Gelatin capsules were selected as the model for the distance test. Gelatin is important to the pharmaceutical industry because of the diverse formulations that can be incorporated into the hard gelatin capsule (HGC). Gelatin capsules are practical containers because of their strong but flexible backbone, polished appearance, capacity to hold dyes, and their solubility in aqueous solutions.4 Gelatin itself is still an occasional source of problems in production processes. For example, a recent batch of gelatin was recalled in December 2001 because it was found to contain an unapproved additive.5 Gelatin is vulnerable to chemical modification, and formaldehyde modification of gelatin has been studied most extensively. Cross-linking of gelatin with formaldehyde has been used to manufacture enteric hard and soft capsules. Exposing gelatin capsules designed for immediate release of their contents to trace levels of formaldehyde may have an adverse effect on their in vitro dissolution rates. Contemporary research indicates that reduced in vitro dissolution rates, in contrast to decreased in vivo bioavailability of drugs, remain the chief consequence of cross-linking of HGCs with low levels of formaldehyde. The literature suggests that cornstarch, a common pharmaceutical excipient that sometimes incorporates a small amount of hexamethylenetetramine stabilizer, may form ammonia and formaldehyde upon hydrolysis and contribute to cross-link formation. The effect of lysine cross-links such as those formed as described above on the dissolution of hard gelatin capsules containing amoxicillin is typically quantified 45 min after the capsules have been placed in a USP-standard dissolution apparatus. Exposure to 150 ppb formaldehyde gas drops the percent amoxicillin dissolved in such tests from 90 after no exposure to 20 after 8 h exposure to the formaldehyde. Previous literature indicates that these lysine cross-links have signals in the near-IR spectral region from 5555 to 5880 cm−1,4 so this spectral region was scanned in the distance experiment. The spatial resolution of remote hyperspectral imaging and number of samples that can be tested simultaneously was tested with tablets in blister packaging. Like capsules, pharmaceutical tablets also periodically have problems that can be detected by hyperspectral imaging. For example, in February 2002 Trandate, a drug indicated in the management of hypertension, was recalled because of dissolution failure at stability testing.6 Trandate was available in 100 unit dose packages (10×10 tablets). Polymer blister covering is a common method of packaging drugs for unit dosing. In this experiment, aspirin was selected for study because its breakdown mechanism is well understood. Aspirin tablets can be placed in a blister pack so that breakdown can be initiated easily by punching a small hole in the package and putting the package into a hydrator. Thousands of aspirin tablets can be inexpensively packaged for hyperspectral imaging using a near-IR camera. Near-IR cameras are also being employed increasingly in hyperspectral imaging experiments. Imaging spectrometers based on array cameras have fast scanning ability and high sensitivity. This image fidelity study tests the hypothesis that a near-IR camera and hyperspectral imaging permits the identity and composition of large numbers of tablets to be determined simultaneously through blister packs to monitor drug stability. 2.Instrumentation2.1.EquipmentA 10 mW helium–neon (HeNe) laser (Spectraphysics, Eugene, OR) was used as a source of visible light for aligning single capsules and optics. The experiments were conducted outdoors to provide the unobstructed 500 m path, and at night to simplify gross alignment of the optics using the visible laser. A 4 mW external cavity tunable diode laser (EOSI, Boulder, CO) was used as a source of near-IR light. Silicon charge coupled device (CCD) (Nikon) and indium antimonide (InSb) digital cameras (Cincinnati Electronics, Mason, OH) were used during the alignment process. A Stanford Research Systems programmable wave form generator was used to modulate the near-IR light at 1 kHz. A small reflecting telescope with a 9 cm aperture was used to collect light for detection. A PbS telescopic detection unit7 was temporarily borrowed from the 1 m near-IR telescope at the university to detect the modulated light from the capsules at 500 m. A Dell PC and 16 bit analog-to-digital converter (A/D) were employed for data collection. Digitized signals from the telescope preamplifier were cross correlated with the signal from the programmable function generator, and Fourier transformed using GLIB software in Mathematica (Wolfram Research, Champaign, IL). To reduce the total data-collection time, signals were recorded at six different wave numbers, 5797, 5764, 5731, 5682, 5634, and 5618 cm−1. The laser samples less than 1 mg of a 60 mg gelatin capsule without moving the position of the beam. Precisely aligning the invisible near-IR laser following initial alignment with a visible laser would have been very difficult without interchangeable precision micrometer mounts. These mounts were constructed for this study in the machine shop of the Department of Physics and Astronomy. The physical length of the optical path was 250 m. A 500 m total optical path was achieved by reflecting the signal from the capsules at 250 m backward using a 20 cm×25 cm first-surface aluminized mirror (see Figure 1). The distance from the laser to the capsule was 2 m, although the beam spread was low enough to permit active excitation of the capsules with the laser at distances of up to approximately 200 m. Figure 1A schematic diagram showing the relationship of the instruments and optical path during collection of spectra at a distance of 0.5 km from a single gelatin capsule. ![]() An IRC-160 InSb focal plane array video camera (Cincinnati Electronics, Mason, OH) with near-IR bandpass cold filter was used for 0.5 m hyperspectral imaging of the samples. The camera frame rate was 51.44 frames/s and the photon energy response was 1800–10 000 cm−1. A polarizing filter for the camera reduced specular reflectance (Polaroid, Cambridge, MA). A tunable interference filter unit8 with photon energy response from 4325 to 5960 cm−1 was mounted on the camera. A Mitsubishi IR-M300 PtSi 256×256 CCD array camera (Cypress, CA, USA) was used at 2 m on the samples. The light source was two 250W PC37771 near-IR/IR lamps (General Electric, Cleveland, OH). A monochromator system employing a concave holographic grating (American Holographic, Fitchburg, MA) and lead sulfide (PbS) detector was used for reference spectra.8 Whenever a near-IR camera was used to collect tablet spectra in blister packages, two spherical silicon dioxide reflectance standards (one high reflectance, and one low reflectance) were placed in each image to control for variations in light intensity and direction. Images collected on different days and with the light sources in different locations were made comparable by adjusting the gain and offset by multiplicative scatter correction9 on the images so the intensities on the standards were identical. The specular reflectance on the standards was used to pin down the locations of the light sources and to supply a method to calibrate reflected specular light intensity. Diffuse reflectance from the rounded surfaces of the two standards was used to calibrate shaded areas and oblique surfaces in the images.10 Two light sources were employed for spectrometric imaging to diminish shadows and attain the greatest signal-to-noise ratio (S/N) possible. The light sources were positioned at 90° relative to one another, with the tablets at the vertex. The camera was placed between the light sources, at an angle of 45° to each. Spectral images were obtained at each wave number with the near-IR light sources turned off, and again with them turned on, to correct for the existence of other lights in the room and for blackbody photon emission from the sample. A hydrator was assembled to control the conditions under which the tablets decomposed.1 Tablets in blister packages were exposed to water vapor or a pH 9.0 ammonium hydroxide solution by punching a hole using a center punch through the foil support. Water absorption was determined by weighing and by near-IR spectrometry. The high-performance liquid chromatography (HPLC) for analysis of salicylic acid used a C-18 column (Analytical Sciences, Santa Clara, CA), a Prostar 320 absorbance detector (Varian, Palo Alto, CA), a model 215 pump (Varian). The software for the near-IR equipment as well as the chromatography interface was written in C++ (Microsoft, Redmond, WA) and Speakeasy (Speakeasy Computing Corp, Chicago, IL). The mobile phase was 27:73 methanol:water, 0.15 M NaH 2 PO 4, 5 mM tetrabutylammonium phosphate, at pH 6.0. This pH was selected to make the results comparable with our earlier study.1 The half-life of aspirin at pH 6.0 is more than five days. Retention times for aspirin were approximately 5 min. The tablet masses were measured with an electronic balance. Tablets were dissolved for HPLC analysis. A total of 43 tablets were selected from 172 hydrator tablets by principal component analysis (PCA) selection algorithm.11 The benefit of this methodology is that it selects the best tablets to use in the calibration based on their near-IR spectra before reference measurements are obtained. The near-IR technique is fast and easy compared to the HPLC assay, making the selection of the optimum subset of tablets for the reference assay based on near-IR spectra valuable. The 172 tablets in turn came from four batches of 43 tablets, each sequence run for up to 24 h in the hydrator.1 By staggering the starting times of each 24 h cycle it was possible to get at least six tablets in each hour of exposure from 0 to 24 h. The four runs were made over an interval of two weeks. 3.MaterialsGelatin capsules (size 2, clear) were obtained from Lilly (Indianapolis, IN). The dissolution medium consisted of 0.2 wt/v sodium chloride and 0.7 v/v conc. HCl in distilled water. Formaldehyde, 37 wt solution in water (stabilized with 15 methanol) was purchased from Aldrich, Milwaukee, WI. The formaldehyde reagent consisted of an aqueous solution of 2.0 M ammonium acetate (98, Aldrich) and 0.020 M 2,4-pentanedione (>99, Aldrich), adjusted to pH 6.0. The amoxicillin reagent consisted of 464 ml of 0.10 M citric acid and 536 ml of 0.20 M disodium hydrogen phosphate. The pH of this reagent was adjusted to 5.20 with citric acid or phosphate buffer. Fifteen ml of 0.0158 M copper sulfate pentahydrate (98, Spectrum, Gardena, CA) was diluted to a volume of 1l with the pH 5.20 citric acid–phosphate mixed buffer. Amoxicillin trihydrate used for filling the stressed HGCs was Amoxil® (SmithKline Beecham, Philadelphia, PA) brand formulation, while amoxicillin trihydrate (99 pure) utilized for preparation of standards was obtained from Sigma (St. Louis, MO). Near-IR images for spatial resolution and sample number tests were obtained from 325 mg aspirin tablets (Kroger, Cincinnati, OH). Reference spectra were obtained from blister packaging (AH Robins, Richmond, VA), water, an aspirin tablet, and salicylic acid (Sigma). Aluminum foil backing (Reynolds, Richmond, VA) and cement (DAP, Dayton, OH) were used for sealing the polyvinyl chloride (PVC) blister packaging. Tetrabutyl ammonium phosphate (Eastman Kodak, Rochester, NY), monosodium phosphate monohydrate (Fisher, Pittsburgh, PA), ammonium hydroxide (Fisher), and methanol were used for the reference HPLC analysis of salicylic acid in aspirin. Part of the S/N problem with focal plane arrays arises from inactive (“dead”) pixels and “flickering” pixels. Some inactive pixels merely generate no output. However, inactive pixels often produce a stable signal beyond the typical range of values from normal pixels. The inactive pixel signal value does not change with a varying optical signal. Manufacturers commonly incorporate software corrections for these pixels into their equipment. These corrections automatically replace the signal values of the inactive pixels with the signal values of adjacent pixels. If a camera performs such an inactive-pixel correction automatically on booting without informing the operator, it can cause errors, especially when analyzing arrays of tablets in blister packaging. When a large number of tablets is in the field of view, only a few pixels are on each tablet; in these cases, it is easy for most or all of the values on an individual tablet to be inaccurate. If the correction software cannot be circumvented, one might never know that a tablet reading is absurd. For this reason, access to raw data from the camera (as used in this study) is preferred over corrected data. Flickering pixels have a signal that varies randomly and partially independently of the actual optical signal. This characteristic makes flickering pixels much harder to correct reliably with automatic routines in actual camera use, because the varying signal could be real. In addition, if the system averages frames to generate a final image, then elementary averaging can obscure the flickering. Flickering pixels are usually not corrected in camera software. Imaging reference cards or scenes (in which the pixel output variation is known) at the beginning of camera operation will detect these pixels early, however. It is notable that flickering pixels frequently become inactive pixels with time. Approximately 0.1 of pixels in an InSb focal plane array are ordinarily inactive or flickering, but this number grows slowly with thermal cycling and age of the focal plane array. 3.1.ChemometricsPrincipal component regression is usually accomplished with a transformation of spectra to principal axes, followed by ordinary least-squares regression. Median-based regression models such as least median of squares are a convincing alternative to mean-based regression models when the distribution of errors is unknown and likely to be non-Gaussian. Remote sensing measurements with an invisible near-IR laser are especially prone to experimental errors that have heavier tail density than the Gaussian distribution. Sample heterogeneity and alignment problems can manifest themselves as clusters of larger residuals in a robust principal component regression. Most approaches to median regression are algorithmic and the advantageous properties arise only asymptotically. Nonparametric error depictions are often the foremost models to apply in the case of smaller sample sizes (i.e., fewer calibration spectra) where parametric specifications are hard to assert. A Bayesian method that implements accurate inference when supplied with the observed spectra is especially attractive in such cases.12 Median-based regression is optimal just for describing skewed and unusually shaped error distributions, and such distributions can be obtained using mixtures of families of error distributions that have the accessible prior probability functions needed for Bayesian inference. The variety of mixture densities defined in Ref. 12 allows skewness and greater variability to be properly accounted for in regression models. Furthermore, the form of the kernel of the mixture permits unambiguous quantification of skewness via a parameter denoted γ. However, the class embodying the densities f(⋅;G,γ) is merely a subset of the class F={f:f unimodal density on R with η(f )=M(f )=0}, where η(f ) and M(f ) signify the median and mode of f, respectively. The model of Kottas and Gelfand depicts all possible densities in F,12 accomplished by designating nonincreasing densities on [0, ∞) as mixtures of uniform densities. To be precise, any nonincreasing density g on [0, ∞) can be expressed as where G is a distribution on [0, ∞). Others have used this result,13 and, embracing a Dirichlet process prior for G, achieved Bayesian semiparametric inference for symmetric unimodal densities. In another example, Brunner utilized the result in modeling the error distribution in linear regression.14Kottas and Gelfand12 employed two nonincreasing densities of the form in Eq. (1) to obtain a general depiction for a density f in F, one utilized for [0, ∞) and the other for (−∞, 0), producing where −[θ1]<·<[θ2], and G1, G2 are distributions on the interval [0, ∞). Of course, any density in F grants the depiction in Eq. (2), because for each of F’s pieces on the negative and positive axes, the portrayal in Eq. (1) is put to use. The case G1=G2 in Eq. (2) furnishes the subclass of symmetric and unimodal densities on the real line.13 14The free type of the density f(.;G1,G2) obviates many analytic results regarding properties of the allied distribution.7 Fortunately, the analytic properties can be connected to the corresponding properties of the mixing distributions G1 and G2. For example, the expected value and the skewness functional γη are given by 1/4[∫θG2(dθ)−∫θG1(dθ)] and (∫θG2(dθ)−∫θG1(dθ)]/[∫θG2(dθ)+∫θG1(dθ)], respectively, as long as G1 and G2 have a finite first moment. If η(G) denotes the median of Eq. (1), then γq(G1,G2)=[η(G2)−η(G1)]/[η(G2)+η(G1)]. When applying Eq. (2), independent Dirichlet process priors are utilized for G1 and G2. The posterior of γq(G1,G2) can be perfected through the posteriors of the quantiles that determine it.12 Separate mixing distributions can be used to control the behavior of each of the tails. Skewness can be described by more than a single parameter, enabling description of more sophisticated forms of asymmetry. However, in certain applications, such added flexibility can exaggerate the data, leading to unstable inference. Models must be selected according to context.12 Nevertheless, the application of robust median-based regression to spectra prevents leverage effects in ordinary least-squares regression from masking experimental variables, which can lead to an increase in overall prediction error. 3.2.Tablet Imaging with the Bootstrap Error-adjusted Single-sample Technique (BEST)The bootstrap error-adjusted single-sample technique (BEST) calculates distances in multidimensional asymmetric nonparametric central 68 confidence intervals in spectral hyperspace (approximately equivalent to standard deviations). The BEST metric can be thought of as a “rubber yardstick” with a nail at the center (the multidimensional mean).15 Because of the nail, the stretch of the yardstick in one direction is independent of the stretch in the other direction. This independence enables the BEST metric to describe odd shapes in spectral hyperspace (spectral-point clusters that are not multivariate normal, like the calibration spectra of many biological systems). BEST distances in standard deviations (SDs) can be correlated to sample composition to produce a quantitative moisture or degradation-product calibration in tablets, or simply used to identify regions with moisture or degradation product distributions similar to the calibration data in a spectral image. The BEST automatically detects samples and situations unlike any encountered in the original calibration, making it more accurate in such applications than typical regression approaches to near-IR data analysis. The BEST produces accurate distances even when the number of calibration samples is less than the number of wavelengths used in calibration, in contrast to other metrics that require matrix factorization. The BEST retains the direction vector of a standard deviation in hyperspace throughout all calculations, an essential characteristic for multicomponent quantification of sample composition. The BEST calculates the integral of a probability “orbital” in hyperspace by starting at the center of the orbital and working outward in all directions at a uniform rate. The distance between the center of a calibration set orbital and a sample spectrum is proportional to the concentration(s) of the calibration set constituent(s) responsible for the vector connecting the central and sample-spectral points. The direction of the vector identifies the constituent(s) of the calibration set. The BEST direction and distance are often used to create color contour plots of the spatial distribution of moisture or degradation products. In such plots, the contours are drawn at sequential distances in SDs, and red-green-blue colors are used to denote class membership based on vector direction. The intensity of the color is proportional to the amount of substance present. Shades of blue are used to represent sample spectra similar to those already in the calibration set, while shades of red are used to represent sample spectra that contain the selected analyte. Shades of green are used to represent a second analyte or possible interfering effect. The BEST performs well as an assimilation method (a method that progressively increases its analytical performance by incorporating previously unknown samples into its calibration). The calibration samples are analyzed by another reference method in the same manner that Beer’s law is used to develop a conventional spectrophotometric calibration. In the BEST, a population P in a hyperspace R represents the universe of possible spectrometric samples (the rows of P are the individual samples, while the columns are the independent information vectors, such as wavelengths or energies). P * is a discrete realization of P based on a calibration set T, which has the same dimensions as P * and is chosen only once from P to represent as nearly as possible all the variations present in P. P * is calculated using a bootstrap process by an operation κ(T), and P * has parameters B and C, where C=E(P) and B are the Monte Carlo approximation to the bootstrap distribution. The expectation value, E(), of P is the center of P, and C is a row vector with as many elements as there are columns in P. Each new sample spectrum X is analyzed by an operation ψ(T,B,X,C), which projects a discrete representation of the probability density of P in hyperspace by many–one mapping onto the vector connecting C and X. X and C have identical dimensions. The directional standard deviation (SD) is found from the projected probability density in Eq. 3 The integral over the hyperspace R is calculated from the center of P outward. The calculation of a skew adjusted is based on a comparison of the expectation value C=E(P) and C T=med(T), the median of T in hyperspace (with the same dimensions as C) projected on the hyperline connecting C and X in Eq. (4).The result of the corrected projection is an asymmetric σ that provides two measures of the standard deviation along the hyperline connecting C and X Equation (5) is in the direction of X in hyperspace, and Eq. (6) in the opposite direction along the hyperline connecting C and X. Skew adjusted SDs can be used to calculate mean distances between spectra of different samples.The use of these equations in both quantitative and qualitative analysis of samples provides a number of advantages over all other methods of analysis. 1. No analytical assumptions are required to make the problem solvable. Other chemometric methods typically assume that no discriminating variable (wavelength) is a linear combination of other discriminating variables, that the covariance matrices for all spectral groups are approximately equal, and that each group is drawn from a population that is normally distributed on the discriminating variables. None of these assumptions is usually true and violations of these assumptions increase the likelihood of producing incorrect quantitative and qualitative analytical results. 2. This nonparametric assimilation method can be used with full spectra of samples, which often include thousands of variables that describe each sample. The large global memory on the newest supercomputers has made possible the manipulation of images involving millions of spectra at thousands of wavelengths. No wavelength selection procedures or data compression techniques, such as principal-axis transformation or Fourier transformation, are required by this assimilation method in order to analyze complete spectra. Collinearity in the discriminating variables (wavelengths) does not degrade the results. (Collinearity disrupts conventional matrix techniques like the Mahalanobis method, which relies on matrix factorization to produce a distance in SDs.) 3. The vector CX in the assimilation method identifies the sample components. The metric is calculated by a highly parallel code that can be distributed across as many processors as are available, and can be used in computerized searches of spectral libraries for qualitative analysis of real samples. The length of the vector is proportional to the concentrations of the constituents. Thus, quantitative analytical capabilities are also provided by the same assimilation method, which can still be distributed across as many processors as are available. 4. The BEST metric is not only more accurate and precise than the Mahalanobis metric (the metric commonly used in near-IR spectrometry), the BEST metric is often calculated more rapidly as well. The matrix inversion required by the Mahalanobis metric is usually accomplished by algorithms whose complexity (in terms of number of operations required) increases as the number of wavelengths cubed. In contrast, the complexity of the BEST metric increases linearly with the number of wavelengths. The assimilation model developed by the BEST can be compressed if desired with singular value decomposition procedures to conserve memory. The model can also be converted into a hash table and hash function by calculating the distance and direction from the center of the calibration set to each of the replicates, and then used in ASIC microchips or on laboratory PCs. 4.Results and Discussion4.1.Distance StudyFigure 2 shows the bright visible and near-IR images of the capsules on the 500 m path. The intensity of the signals received suggests that spectrometry over even greater distances is possible. Spectra were collected individually from 24 hard gelatin capsules. Six of these capsules were not exposed to formaldehyde, while six were exposed to 150 ppb formaldehyde for 2 h, another six for 4 h, and the last six for 8 h. Principal component regression of the spectra versus exposure time to formaldehyde produced r2=0.89, with the standard error of estimate (SEE)=1.17 h and SEP=1.17 h. The calibration line is shown in Figure 3. The process of cross-linking in gelatin capsules is not homogeneous, and cross-linking tends to occur most extensively in the portions of the capsule closest to the source of moisture and aldehyde, where the concentrations are highest. The laser beam is relatively narrow in comparison to the capsule. The r2 would probably have been higher if the entire capsule had been illuminated by the laser source and analyzed spectrometrically instead of less than 1 mg of the sample (previous full-capsule experiments in the laboratory yielded r2=0.963 ).4 In addition, a smaller wave number range was scanned than is usually employed for capsules to save the time required to change laser heads for extended wave number range. Finally, because the experiment is conducted outdoors, some low frequency noise is introduced in the system by wind. Most of the wind-induced motion occurs in the distant mirror, which is supported by a tripod. A 20 lb sandbag was hung from the tripod to reduce motion and the chance that the tripod would blow over in an elevated location. This solution was not 100 effective in preserving alignment of the invisible near-IR laser beam over extended periods, however. For this reason, robust (median-based) regression was employed to enable detection of alignment problems in the samples. Figure 2The visible light image (top) and near-IR light image (bottom) shows the intensity of the signal received over the 0.5 km path from a single gelatin capsule. ![]() Figure 3The actual vs predicted exposure time to formaldehyde calculated using the spectra acquired at a distance of 0.5 km. ![]() These results suggest that active-excitation spectrometry is a technique that is ripe for further research in pharmaceutical analysis. The active-excitation technique is particularly applicable to closed vessels where passive excitation does not reach. Indeed, NASA’s Jet Propulsion Laboratory is working on a multifunctional active-excitation spectral analyzer (MAESA) for missions to planets like Pluto.16 Pluto is so far from the sun that passive excitation would not be effective. The MAESA uses a laser to illuminate either a point or a line on a target. Raster scanning permits hyperspectral images to be collected. With the addition of a diffraction grating on the detection end of the system, active excitation can also be used to obtain Raman or fluorescence spectra. The NASA device features a wavelength response from 500 to 2500 nm, low power consumption, and a portable package design that operates near room temperature. Figure 4 shows illustrative spectra of an aspirin tablet (dotted line), the blister package (solid line), an aspirin tablet in a blister package (dot-dashed line), and an aspirin tablet in a blister package with a leak exposing the tablet to moisture (dashed line). These spectra were obtained from single samples with the nonimaging spectrometer. The major peaks of the packaging are at 5700–5800 and 4000–4350 cm−1. The major spectral peaks of acetylsalicylic acid, at about 6050 and 4200–4800 cm−1, are apparent through the packaging. The most distinguishing spectral feature of salicylic acid arising through aspirin decomposition appears at 6370 cm−1.1 The major water-absorbance peak appears at approximately 5200 cm−1. This section of the spectrum contains only modest interference from the packaging, which simplifies the imaging of tablets. Calibration lines were computed for water and salicylic acid. The calibration lines were created using data obtained at 0.5 m from 43 tablets selected from 172 by the PCA method of Svensson, Josefson, and Langkilde.11 The standard error of estimate (SEE) for water was 0.05 of tablet mass and the SEP was 0.06 of tablet mass (r2=0.96). For salicylic acid, the SEE=0.06 of tablet mass and SEP=0.06 of tablet mass (r2=0.98). The correlation is calculated between the concentrations predicted from near-IR spectra and the concentrations determined by reference methods, i.e., weighing (for water) and HPLC (for salicylic acid). Figure 4Representative spectra of aspirin tablet (dotted line), the blister package (solid line), an aspirin tablet in a blister package (dot-dashed line), and an aspirin tablet in a blister package with a leak exposing the tablet to moisture (dashed line). ![]() The PC (principal component) plot in Figure 5 portrays water uptake by ten packaged tablets in the field of packaged tablets shown in the contour plot in the inset. The tablets were imaged at 8, 16, and 24 h after a hole was punched in the foil backing. The times are marked on each tablet in the plot. The end point was at the same time for all tablets, and the starting time (i.e., when the hole was punched) was varied. The tablet spectra were collected through the blister packaging, and the spectra are shown after multiplicative scatter correction. The spectra were used to calculate the contour plot in the inset of Figure 5. The contours connect pixels with the same distance in multidimensional standard deviations (SDs) from the calibration tablets centered near the origin in the PC plot. The PC scores of the spectra show that the changing signal from water over time is readily detected through the blister packaging using a near-IR camera. In Figure 5 a distance less than 3.8 SDs from the calibration set of normal tablets is displayed as light gray contour. A distance greater than 3.8 SDs in the direction of the spectrum of water (shown by the arrow) is colored progressively darker. The intensity of the black color is correlated to the magnitude of the distance in SDs between each pixel spectrum and the center of the spectra of control (dry) tablets. The maximum distance is 8.6 BEST SDs (corresponding to the tablet with the blackest density in the contour plot, and highest spectral peak at 5200 cm−1). Previous studies in nonpharmaceutical applications have suggested that there might be a minimum number of pixels required on a sample (e.g., 16) to achieve an acceptable S/N.1 Figure 5Contour plot with lines drawn in bootstrap error-adjusted single-sample technique standard deviations (SDs) for ten packaged tablets in a field of 1286 packaged control tablets. Data are not interpolated from the array. Gray contour lines are <3.8 SDs from control tablets. Darker coloration begins at 3.8 SDs and peaks at its blackest at 8.6 SDs. ![]() Figure 5 suggests that the upper limit on the number of tablets that can be imaged concurrently on a 256×256 array is approximately 1300. With 1296 tablets in the camera field of view, at least 16 pixels can be applied in simultaneous sampling of each tablet, which is enough spectra on each tablet to get an effective S/N and form contours for imaging. Principal component analysis of the tablet spectra showed loadings with spectral features that correspond to salicylic acid and acetylsalicylic acid.1 5.ConclusionsAccording to a recent article in the Legal Practice and Management Report,17 manufacturers of medicines and supplements are extremely vulnerable to litigation because the market is so competitive and lucrative that drug companies “rush their products to market without proper testing.” Toxic tort as a legal specialty has become very lucrative as well. With many things that can conceivably go wrong with production, litigators are cashing in on the suspicion that pharmaceutical producers sometimes know or suspect that “they have a faulty product, all the while crossing their fingers that it won’t cause any actual harm.” The ever-growing demand for drugs increases the possibility of defective products reaching the marketplace and, of course, the need for pharmaceutical suppliers to defend against all manner of class-action litigation. In keeping with the common wisdom that the best defense is a good offense, the pharmaceutical industry is making use of evolving technologies that can characterize oral and inhalation drug delivery systems, including tablets, capsules, beads, and inhalant powders. Hyperspectral imaging technology is especially useful to scientists involved in formulation design, formulation development, reformulation, or process analysis. Applying this level of pre-emptive scrutiny to medicine manufacturing is likely to reduce incidences of product defect. In the end, the biggest winner will be the consumer, whose chances of product failure will be lessened by hyperspectral testing. The distance experiment with single gelatin capsules hints how far apart the sample and instrumentation might be while still maintaining adequate S/N. This experiment suggests that remote-sensing technology might some day be profitably applied to cleaning validation and detection of small amounts of materials in a manufacturing environment. The next step is to conduct experiments designed to detect protein contamination of clean glass and metal surfaces by scattering of light. These studies are just beginning. Tests will be conducted with a wavelength selection device (e.g., tunable filter) in front of a camera, and with a MAESA approach. Positive results would support the use of hyperspectral imaging and remote sensing to validation of cleaning in GMP processes. In the image fidelity and sample number experiment, hyperspectral imaging presents a potential speed improvement of approximately 30 000 over HPLC when analyzing moisture and salicylic acid in single, packaged aspirin tablets. Although the multiple-tablet imaging method is not quite as precise as spectrometric analysis of single tablets, the precision is close.1 Hyperspectral imaging of a field of tablets is approximately 1000 times more rapid than spectrometry of single tablets. A near-IR camera including BEST image-analysis software permits multiple tablets to be analyzed simultaneously in blister packages. Further improvements in precision and sample throughput may come with:
Applications of conventional near-IR spectrometry are published almost every day in the pharmaceutical literature. Imaging and computing technology are changing many areas of scientific research. The next major advance of near-IR spectrometry in pharmaceutical analysis will likely come in the form of hyperspectral imaging, which enables small amounts of materials to be detected at a distance, and immense numbers of samples to be analyzed simultaneously. The distance experiment suggests how far apart the sample and instrumentation might be while still maintaining adequate S/N. The multiple-tablet image-fidelity experiment suggests how many samples can be analyzed simultaneously while maintaining an adequate S/N and pixel coverage on each sample. Together, these experiments suggest that hyperspectral imaging is capable of doing much more in pharmaceutical analysis than it is currently asked to do, and that this technology might some day be profitably applied to cleaning validation and remote sensing of small amounts of materials in a production environment. AcknowledgmentThe authors acknowledge the support of the National Science Foundation through CHE-9257998 and DGE-9870691. A preliminary report on this work was published in the Proc. SPIE Symp. BiOS., 2002. REFERENCES
J. Veverka
,
M. Robinson
,
P. Thomas
,
S. Murchie
,
J. F. Bell III
,
N. Izenberg
,
C. Chapman
,
A. Harch
,
M. Bell
,
B. Carcich
,
A. Cheng
,
B. Clark
,
D. Domingue
,
D. Dunham
,
R. Farquhar
,
M. J. Gaffey
,
E. Hawkins
,
J. Joseph
,
R. Kirk
,
H. Li
,
P. Lucey
,
M. Malin
,
P. Martin
,
L. McFadden
,
W. J. Merline
,
J. K. Miller
,
W. M. Owen Jr.
,
C. Peterson
,
L. Prockter
,
J. Warren
,
D. Wellnitz
,
B. G. Williams
, and
D. K. Yeomans
,
“NEAR at Eros: imaging and spectral results,”
Science , 289 2088
–2097
(2000). Google Scholar
T. B. Gold
,
R. G. Buice Jr.
,
R. A. Lodder
, and
G. A. Digenis
,
“Determination of extent of formaldehyde-induced crosslinking in hard gelatin capsules by near-infrared spectrophotometry,”
Pharm. Res. , 14
(8), 1046
–1050
(1997). Google Scholar
T. Isaksson
and
B. Kowalski
,
“Piece-wise multiplicative scatter correction applied to near-infrared diffuse transmittance data from meat products,”
Appl. Spectrosc. , 47
(6), 702
–709
(1993). Google Scholar
R. J. Dempsey
,
L. A. Cassis
,
D. G. Davis
, and
R. A. Lodder
,
“Near infrared imaging and spectroscopy in stroke research: lipoprotein distributions and disease,”
Ann. N.Y. Acad. Sci. , 820 149
–169
(1997). Google Scholar
O. Svensson
,
M. Josefson
, and
F. W. Langkilde
,
“Classification of chemically modified celluloses using a near-infrared spectrometer and soft independent modeling of class analogies,”
Appl. Spectrosc. , 51
(12), 1828
–1835
(1997). Google Scholar
A. Kottas
and
A. E. Gelfand
,
“Bayesian semiparametric median regression modeling,”
J. Am. Stat. Assoc. , 96
(456), 1458
–1469
(2001). Google Scholar
L. J. Brunner
and
A. Y. Lo
,
“Bayes methods for a symmetric unimodal density and its mode,”
Ann. Stat. , 17 1550
–1566
(1989). Google Scholar
L. J. Brunner
,
“Bayesian linear regression with error terms that have symmetric unimodal densities,”
J. Nonparametric Stat. , 4 335
–348
(1995). Google Scholar
R. J. Dempsey
,
L. A. Cassis
,
D. G. Davis
, and
R. A. Lodder
,
“Near infrared imaging and spectroscopy in stroke research: Lipoprotein distributions and disease,”
Ann. N.Y. Acad. Sci. , 820 149
–169
(1997). Google Scholar
|
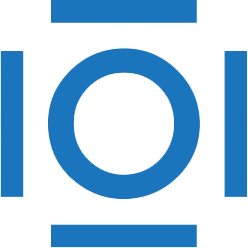
CITATIONS
Cited by 19 scholarly publications and 4 patents.
Tablets
Calibration
Cameras
Hyperspectral imaging
Statistical analysis
Spectroscopy
Packaging