|
ACCESS THE FULL ARTICLE
No SPIE Account? Create one
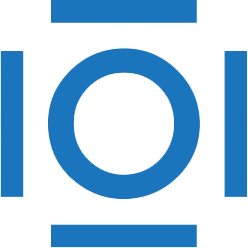
CITATIONS
Cited by 8 scholarly publications.
Feature extraction
Performance modeling
Visualization
Data modeling
Particle swarm optimization
Image fusion
Image segmentation