The vulnerability of face recognition-based unlocking systems to spoofing attacks is a serious problem. With the development of science and technology, face pictures on the Internet are particularly easy to obtain and recreate. A face spoofing attack happens when someone fakes a face and tries to pass the verification of the face recognition system, which seriously threatens the security of users’ information. We propose a method based on quantifying the difference between neighborhood pixels around the center of a local region, namely, weighted neighborhood difference quantization local binary pattern (LBP), against print attacks, and video-replay attacks. The proposed method quantifies the differences between neighborhood pixels without using the center one and uses a linear weighting scheme to improve the discriminant capability of the traditional LBP. The combination of the proposed algorithm and the spatial pyramid further improves the performance of face spoofing detection. We also conducted a lot of experiments in different color spaces to illustrate the role of color in face spoofing detection. The improved method achieves better results in three challenging face anti-spoofing databases, CASIA FASD, Replay-Attack, and Replay-Mobile, respectively. |
ACCESS THE FULL ARTICLE
No SPIE Account? Create one
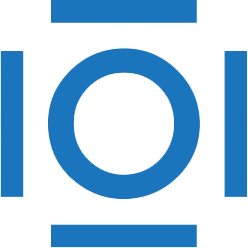
CITATIONS
Cited by 3 scholarly publications.
Databases
RGB color model
Facial recognition systems
Feature extraction
Binary data
Surface plasmons
Detection and tracking algorithms