Compared with the improvement of model structure in the field of object detection, the imbalanced problems in the training process have received less attention, but it is also one of the main reasons affecting its performance. We mainly analyze the imbalanced problems that occur in different stages of the network training process and propose a more balanced network called Balanced-RetinaNet, which has three improvements. First, in the feature extraction stage, a multi-scale feature balanced module is proposed to settle the problem in terms of imbalanced feature distribution, that is, the high-level feature lacks spatial information and while the low-level feature lacks semantic information; then, in the object regression stage, an interval-based regression loss is proposed to solve the problem of inaccurate localization caused by the different contributions of different samples to the regression loss; finally, in the object classification stage, an adaptive focal loss is proposed to solve the problem of classification errors caused by the loss of a large number of negative samples overwhelming the overall classification loss. Experiments have proved that by solving the imbalanced problems, the detection accuracy has been significantly improved on the MS COCO dataset. |
ACCESS THE FULL ARTICLE
No SPIE Account? Create one
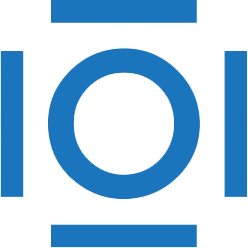
CITATIONS
Cited by 2 scholarly publications.
Feature extraction
Classification systems
Convolution
Detection and tracking algorithms
Image resolution
Performance modeling
Sensors